Mobile app paywall A/B testing: How to get started
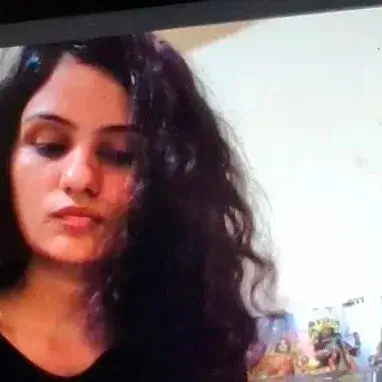
Updated: September 9, 2024
23 min read
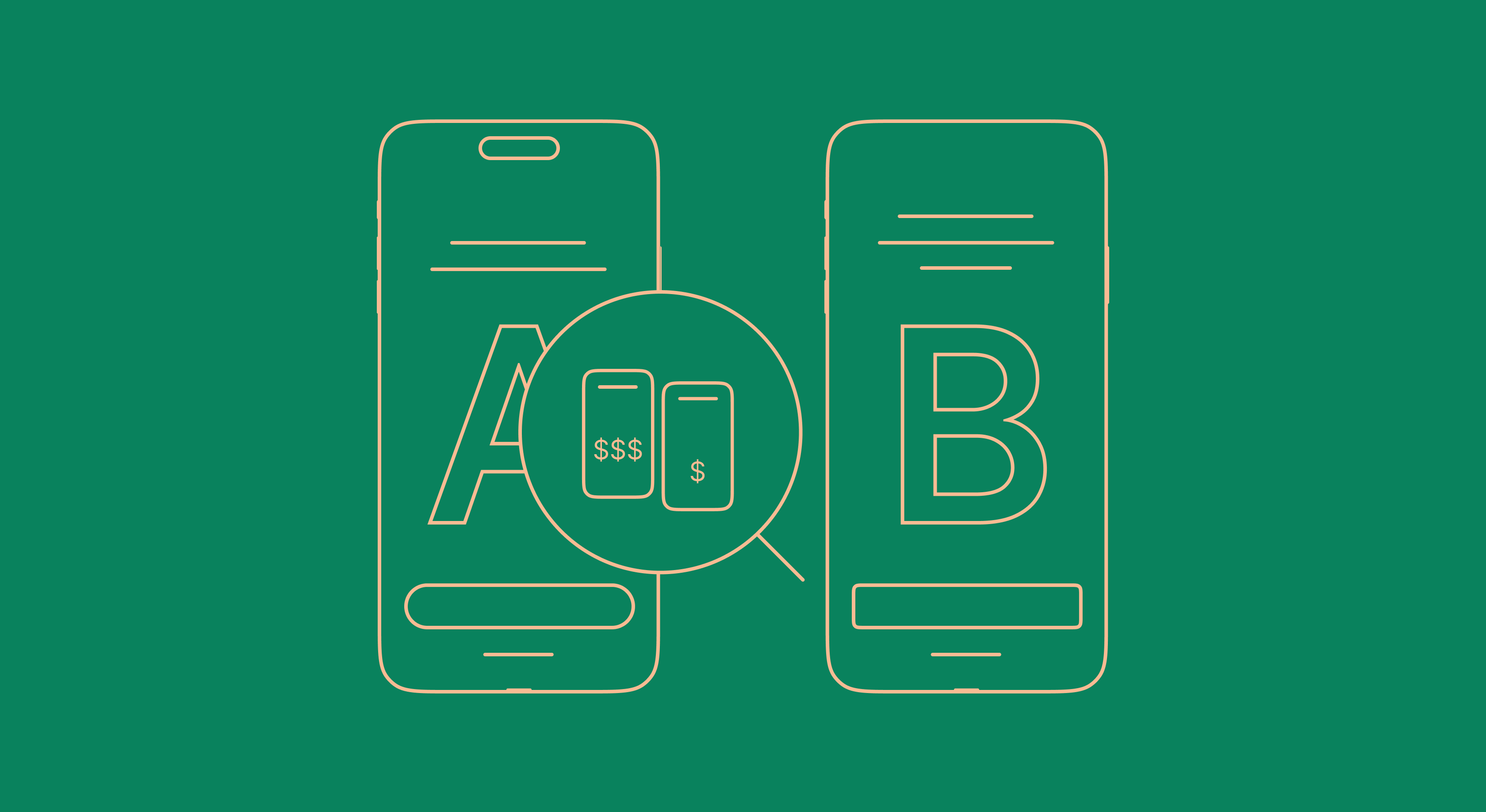
One of the best ways to improve your app business’s bottom line is to begin with paywall optimization.
Paywall optimization – or using experiments to optimize your paywall conversions – helps with both your business’s short- and long-term goals. For example, you can generate some quick capital if you experiment with launching a new offer. By testing different subscription plans, you can redistribute your app’s customer base so that you look at more revenue in the long term. Likewise, experimenting with varying lengths of subscriptions can help you maintain a good cash flow for sustaining your app business.
At Adapty, we power paywall tests for thousands of apps, and we’ve seen businesses reach all kinds of goals via paywall experiments. And we can tell you one thing: Running an effective paywall experimentation program (one that results in continuous and sustainable growth) comes down to running quality experiments.
In today’s article, we’ll see a step-by-step process of how you can plan, implement, and run experiments (that drive real growth) on your paywalls. But first, let’s see a quick primer on paywall A/B testing.
What is mobile app paywall A/B testing?
A mobile app paywall A/B test is an experiment where an app uses two paywalls (“A” and “B”).
Some percentage of the users see paywall A (or the A version). The A version is the original paywall, also called the “control.”
The remaining users see version B (the “challenger”):
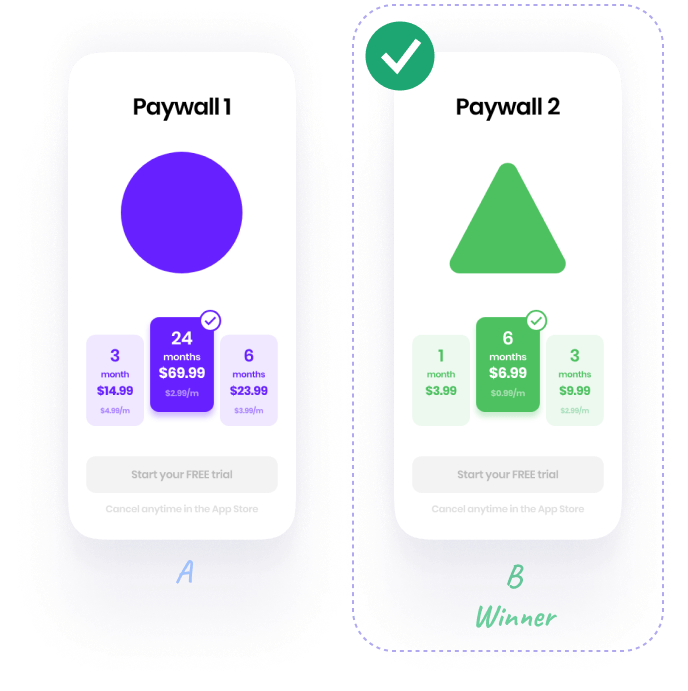
Optimizers observe the performance of both versions, and the one that generates the maximum revenue is the winner. This version gets rolled out for all users at this point.
Using A/B testing, mobile app businesses can optimize their paywall designs, copy, and products (including offers) to generate the most revenue possible.
Let’s now look at a tested 5-step process to help you run winning paywall experiments.
Steps to get started with mobile app paywall A/B testing (We’ll be using Adapty – obviously! – to run our A/B tests!)
- Researching
- Hypothesizing
- Creating test builds
- Testing
- Analyzing
Here’s zooming in on each.
Researching
Researching is the first step to running successful paywall experiments. Using research, you can learn 1) why users convert on your paywall and 2) why they don’t. Paywall A/B testing requires both qualitative and quantitative research.
First comes qualitative research which helps you understand how users feel about your paywall design, copy, and products. This, in turn, helps you test different approaches to these elements to find the ones that connect the best with your audience.
For example, the user testing qualitative research method lets you get “real” users to try your paywall. So if you’ve built a budgeting app for millennials, you’d recruit a group of millennials to check out your paywall and ask them if they think the paywall inspires them to convert. If multiple users report that they find the plans “too expensive,” your pricing may be a problem. You can test newer and more user-friendly pricing plans based on this finding.
Interviewing your actual current “real” users also gives invaluable insights into what got them to take up a trial or subscription or make a purchase on your paywall. If 80% of your newly upgraded users are citing similar responses for upgrading, you can use them to craft a great headline. You can then test it against what you currently use.
Surveys help as well. Surveying new users can highlight your app’s most compelling premium features, and you can try showing them prominently on your paywall to see if they improve your conversion rate.
Qualitative research is an excellent source of ideas to test on an app paywall. But remember that your findings will tie directly to the questions you put to your users. So design a meaningful questionnaire that gives you the insights you need to plan experiments. Here are a few questions to help you get started:
- How much would you pay for this app?
- Which is your most preferred plan of all the available options?
- Would you be interested in a lifetime offer?
- Would you be more likely to subscribe if the app had a trial?
- Will you trust this app – roughly based on the paywall – with your data?
- Describe the paywall’s design in one word.
- Does the paywall explain the benefits of upgrading well enough?
And so on.
Next comes quantitative data.
Quantitative data gives you numbers. It tells you what’s going on on your paywall. For instance, if you use a solution like Adapty to power your app’s paywall, you can collect a lot of data on your paywall. Here are a few key metrics:
Purchase conversion rate: Expressed as a percentage, the conversion rate metric tells you the percentage of users who made a purchase on the paywall. For example, if you had 30 conversions on your paywall and your paywall saw 100 visits, your conversion rate would be 30%. If you see low conversion rates, you might want to start with paywall testing immediately. And what’s a low paywall conversion rate for an app in your industry? Good question. We’re answering it in our Android and iOS reports on subscriptions.
Trial conversion rate: Also expressed as a percentage, the trial conversion metric tells you the percentage of users who signed up for a trial on the paywall. For example, if you had 30 trial signups on your paywall and your paywall recorded 100 visits, your conversion rate would be 30%.
If you have a low trial conversion rate:
- You might want to try a new trial offer copy. Perhaps your current copywriting isn’t so persuasive.
- You might want to offer a trial on a different plan. If you’re letting your users try your annual plan that charges a hefty fee upfront (post the trial), you may want to experiment with running a trial offer on a more budget-friendly plan.
- You may even want to play around with the trial lengths. If you offer a 3-day trial, users might feel it’s not enough and that they’ll be charged too soon.
Alternatively, you might want to use a new trial paywall – like the increasingly popular timeline trial paywall type – that helps sell trials effectively.
Trials canceled: This metric shows the number of users who turned off the auto-renewal.
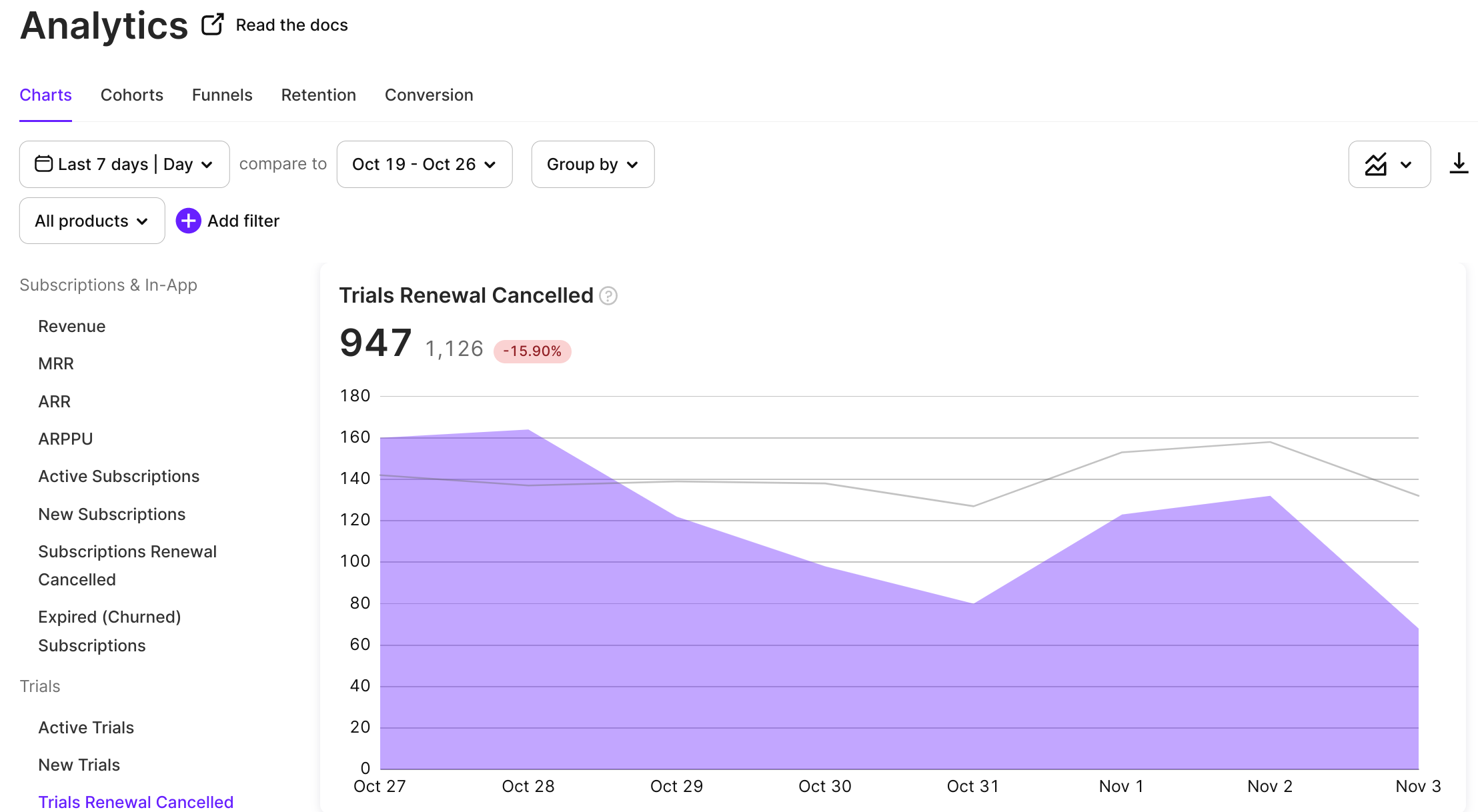
If you observe a lot of cancellations, it may indicate a mismatch between your trial’s offer and the users’ expectations. You could experiment with a different and more straightforward copy. Note that this metric can also hint at deeper issues within your app, like performance.
Heatmap data also counts toward quantitative data. For example, if you’re running a landing page paywall (another increasingly popular paywall type), you might find out via user session replays that users don’t scroll beyond a point. In this case, you might hypothesize testing a shorter paywall or the standard single-screen paywall. User sessions and heatmaps can also show you what your paywall “hotspots” are. These areas get the most attention in your paywall, and you can experiment by adding key pieces of your copy, design, or offer around them. Solutions like Smartlook and UXCam can come in handy for such analysis.
Hypothesizing
The second step of A/B testing your mobile app paywall is using all the data you learned from your research and forming a (data-backed) hypothesis.
Simply put, a hypothesis is a “test” statement that an experiment (an A/B test in our case!) either proves or disproves.
There are three parts to a hypothesis. Let’s quickly go over each:
Observations
Observations are findings from your research.
For example, when the team behind Moonly (an Adapty customer) analyzed its data post a price hike, it observed that users were reluctant to buy the subscriptions at the hiked rates without getting to try the app.
Action
Next are actions. These are things that you *think* will fix the conversion barriers you observed in your data.
In the Moonly app case, the team proposed that offering a trial on the plans would give more people the confidence to subscribe. Also, Moonly decided to provide the trial only on its annual plan (which it wanted to push over its monthly and lifetime subscriptions).
Results
These are the desired results you expect if your observations are correct and the action(s) you decided to fix the conversion issues work as proposed.
For this particular Moonly app paywall experiment, the desired results were improving the paywall’s conversion rate and getting more subscriptions.
Optimizer Craig Sullivan gives a handy template for forming hypotheses. Just fill in your details, and you’re set:
Simple Kit:
1. Because we saw (data/feedback)
2. We expect that (change) will cause (impact)
3. We’ll measure this using (data metric)
If you’re running a more mature experimentation program, your hypotheses can be even more pointed. Here’s Sullivan’s advanced version to help you. As you can see, this one goes beyond the test statement and into how the proposed experiment ties to your business:
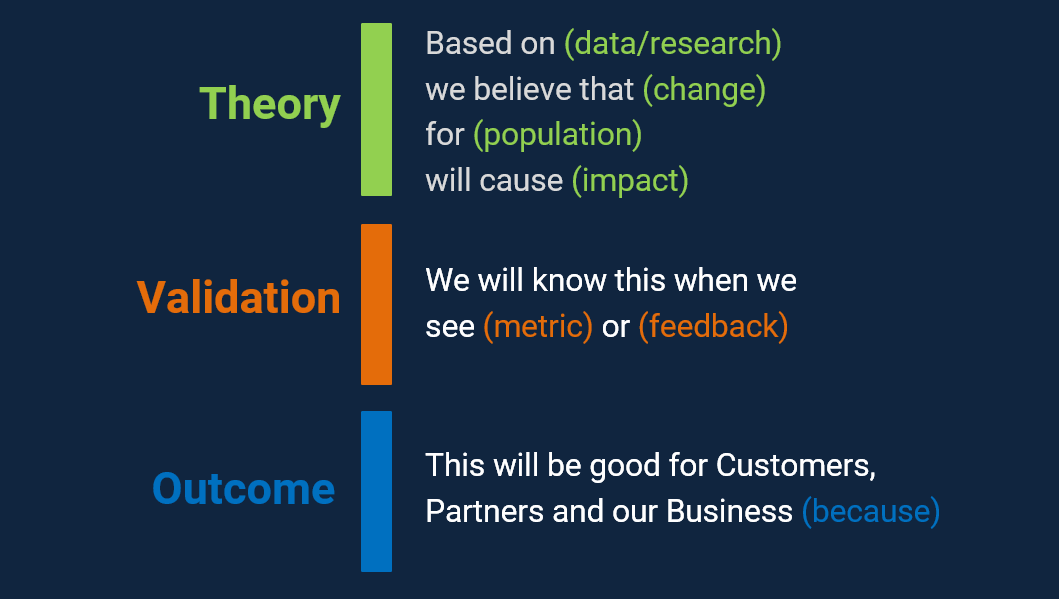
2024 subscription benchmarks and insights
Get your free copy of our latest subscription report to stay ahead in 2024.
Creating test builds
When it comes to paywall A/B testing, you can test almost everything that lives on your paywall. These can be:
- The header area (Banners, sliders, or videos)
- Copy (Headings, subheads, features, benefits, etc.)
- Products (Different pricing plans and subscription lengths)
- Offers (Discounts, trials, etc.)
- The call to action button
- The footer area (Elements like interactive consent checkboxes, links to the terms of use resource and privacy policy, etc.)
To help you get a head start, we’ve rounded up some of the most popular A/B “testable” paywall elements in our earlier post (“What to test on the paywall?”), do check it out. We’ve also covered these elements in detail in our earlier article on the essentials of a mobile app paywall.
Depending on your hypothesis, you’ll need to create a variation of your paywall that fixes the element (or elements) that you think are causing conversion bottlenecks. For example, with Moonly, the lack of a trial potentially resulted in lower conversions. So the experiment was offering a trial. This is how version A (the original or the “control”) and version B looked like for this experiment:
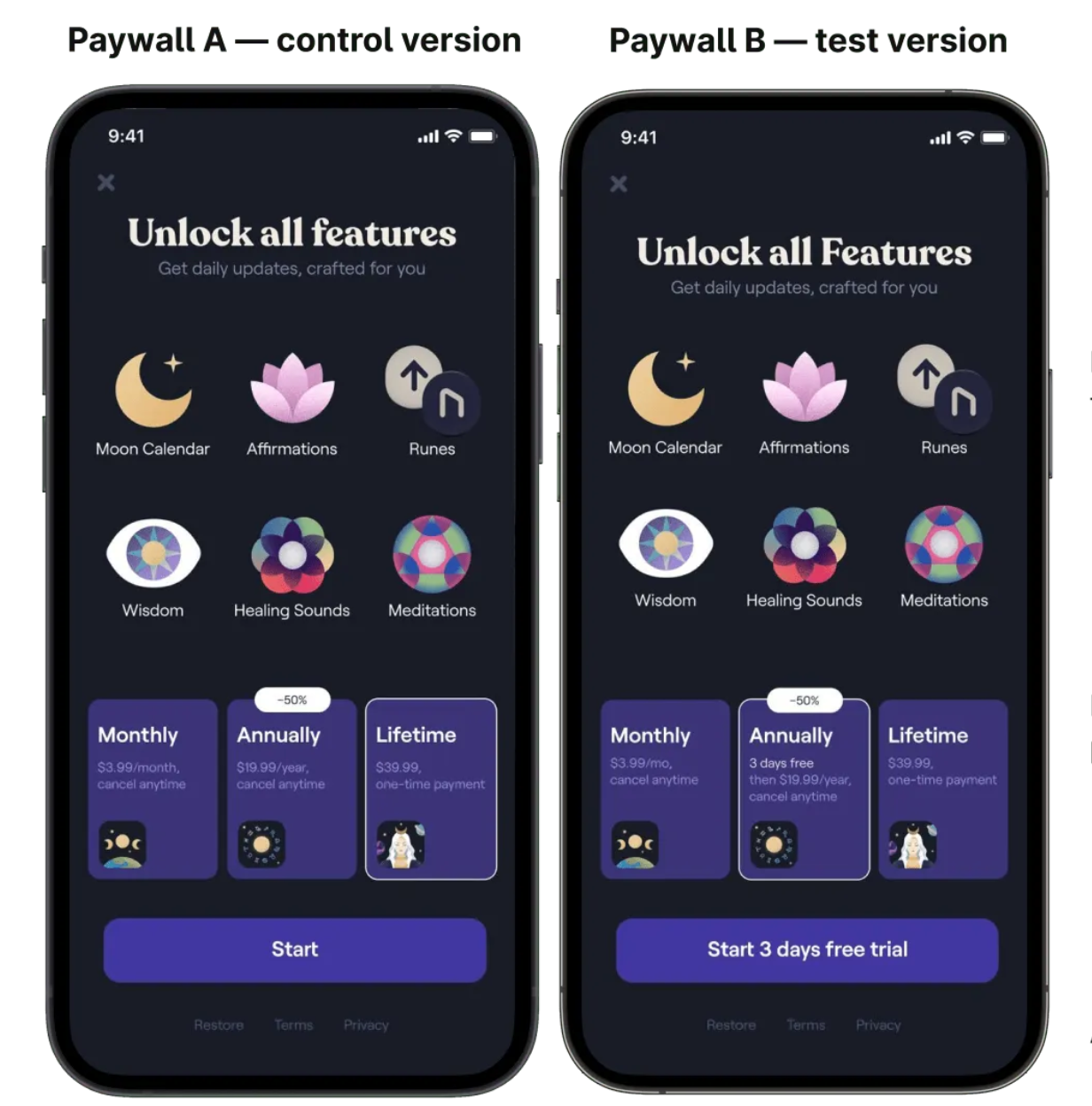
So how do you actually build your test version and add it to your app?
Integrate a mobile app paywall A/B testing solution like Adapty. Here’s how the process of running a paywall A/B test with Adapty works like:
Step #1: Set up your basic paywall
Once your developer integrates the Adapty SDK within your app, it’s time to add your base paywall to your app via Adapty.
To do this, your developer needs to codify all the elements on your paywall and add all the products that you’ve up for sales inside Adapty. These could be one-off purchases or subscriptions. Doing this is easily possible with Adapty. And our support heroes are on standby to offer any assistance you need with this step.
Once this is done and you hit “Save & publish,” your “base” paywall is up and running inside your app.
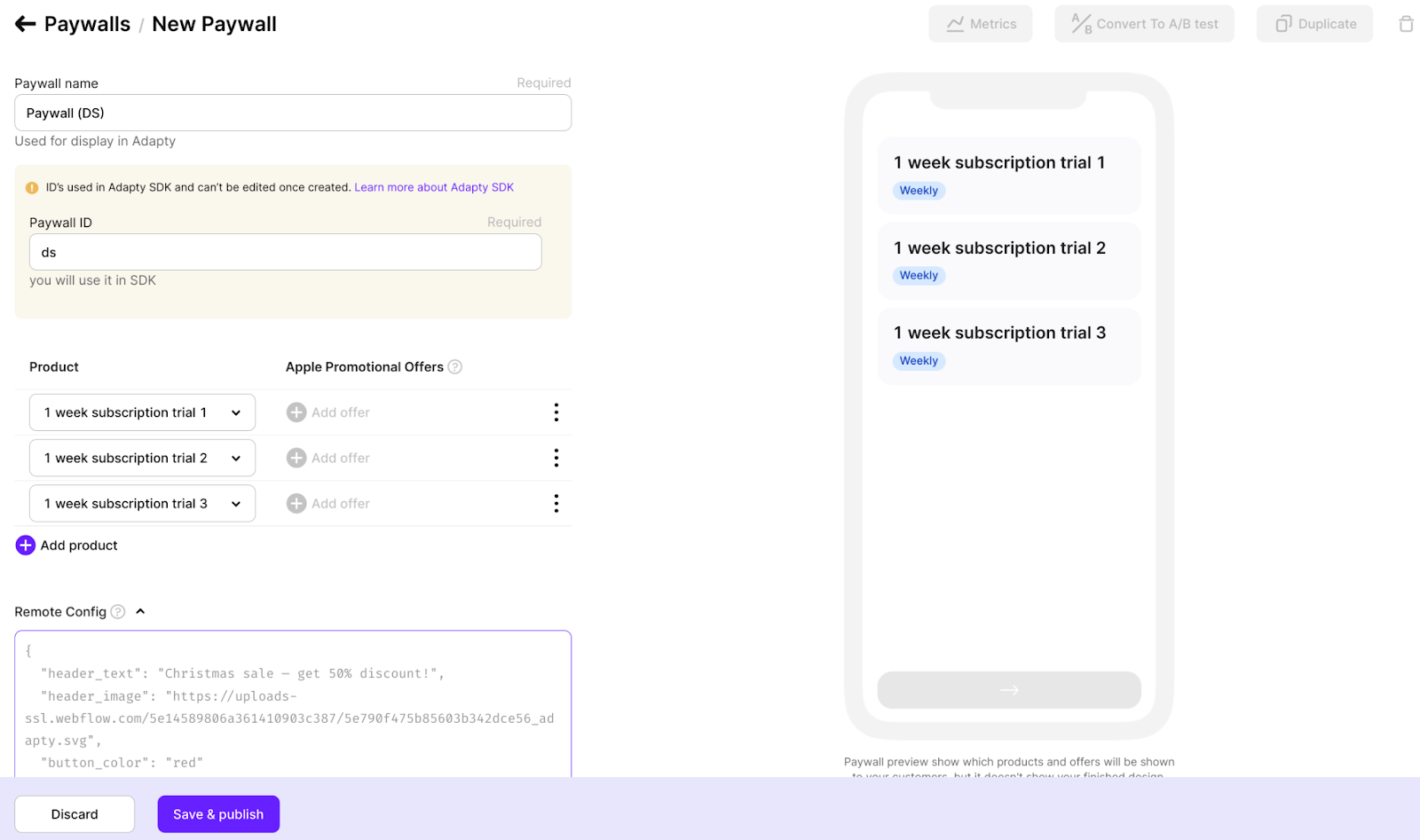
Step #2: Setting up the test
Once your basic paywall is live in your app via Adapty, it only takes two clicks to start setting up an A/B test on it. To do so, access the paywall you want to run a test on and click the options icon and select the “Convert to A/B test” item:
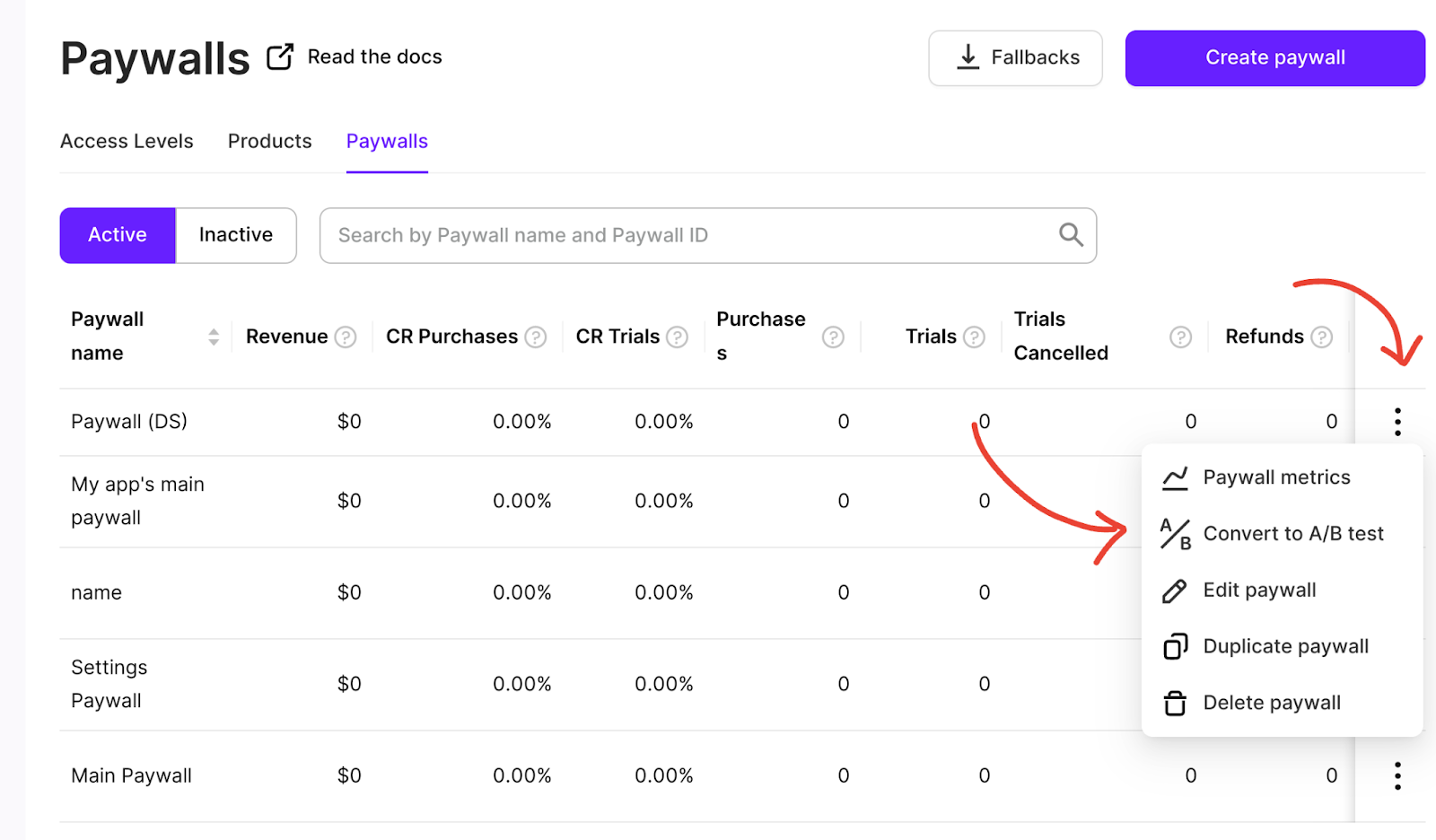
Adapty now asks you to:
- Name your A/B test.
- Set a test ID. Your test ID just acts as an identifier for the paywall being added.
- List your goals with the test. (Anything you hypothesized in the earlier step goes in the “Describe your experiment plans and goals” section.)
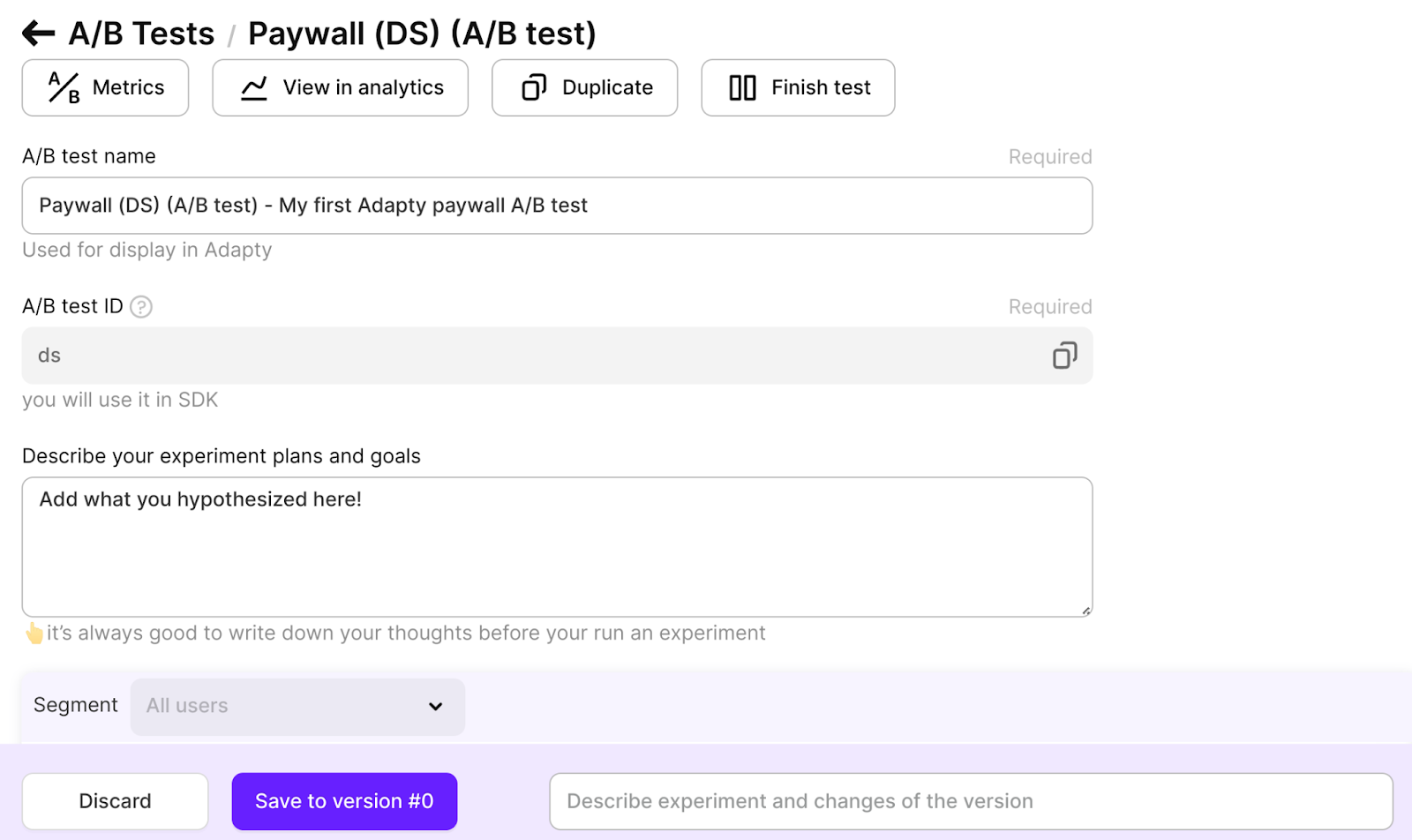
Since an A/B test needs at least two paywalls, you need to add one more paywall to this experiment that uses your basic paywall as the first version.
To do so, scroll down, and you’ll see the option to “Create Paywall.” Click that, and you should see the editor where Adapty asks for details for your second paywall (version):
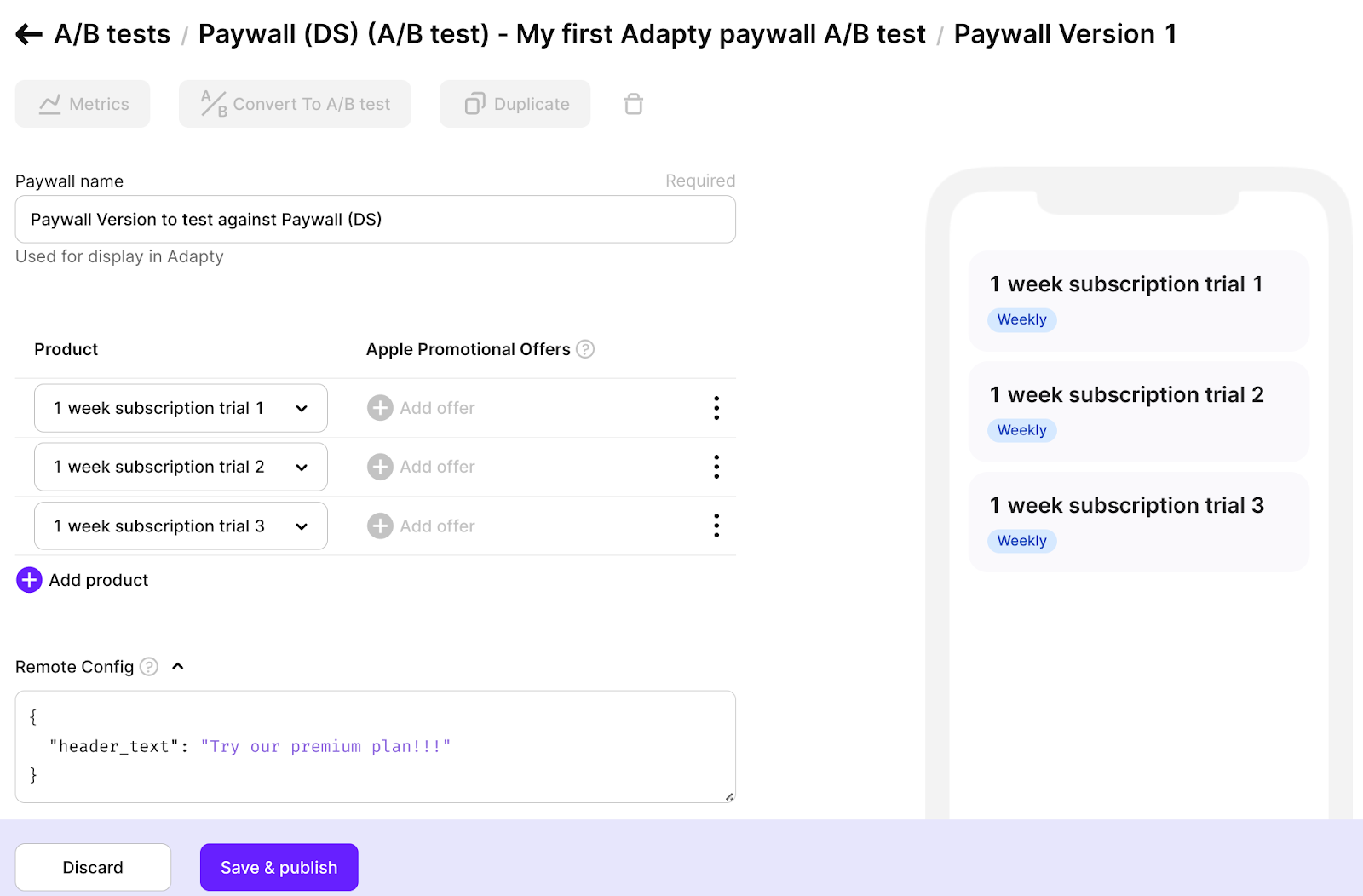
Once you hit “Save & publish,” both the versions of the paywall show up inside your experiment:
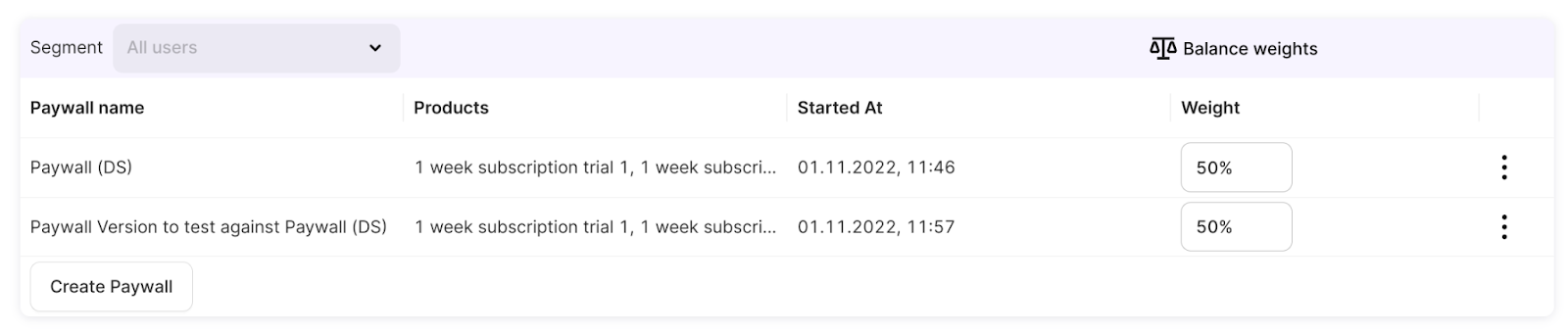
(By default, Adapty assigns a 50-50 traffic split to both the versions. See the weight field for reference. More on this below.)
Step #3: Editing the element(s) to test
When you codify your paywall with Adapty, all your paywall elements become editable via Remote Config. So building your test build means editing the element(s) using Remote Config. Below, you can see how easy running a test on the header is. As you can see, 1) the header image, 2) the headline copy, and 3) the list of features can be edited on the fly:
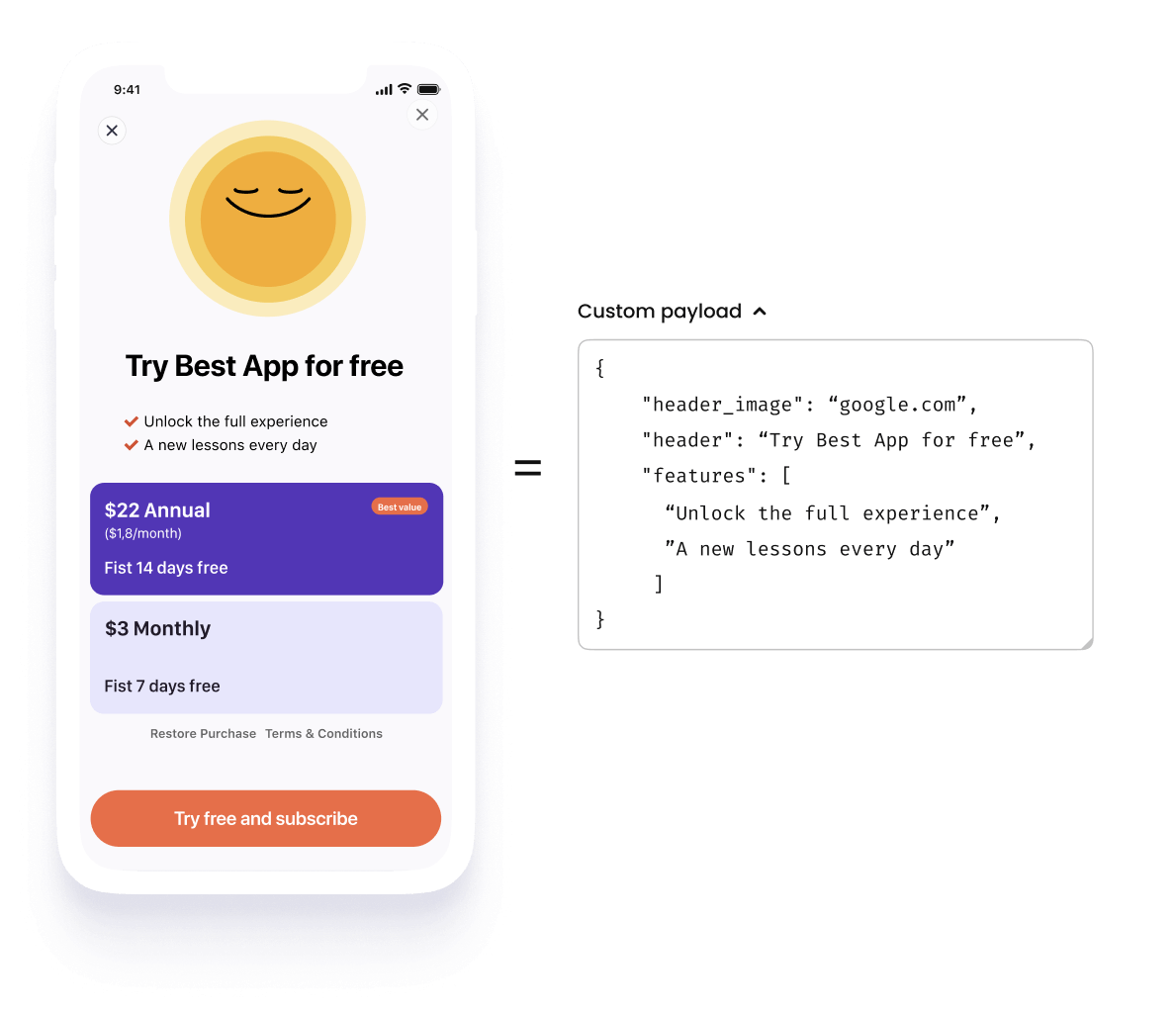
Now, while you can test multiple elements simultaneously, it’s not advisable. Why? When you experiment with more than one thing on the paywall, it’s impossible to definitively tell which change leads to the results. For example, what would you attribute an increase in conversions to if you change your subscription plan’s pricing and your CTA button copy in a single experiment?
It’s best to test one element at a time.
That said, it will work just fine if you want to try two different paywalls altogether.
Testing
When running a paywall experiment, the most important thing is ensuring that you produce statistically significant results. A statistically significant experiment is one where you can be sure that the results your experiment yielded are actually present and that they aren’t any chance development.
If your test hits 95% statistical significance, it means you’re 95% right about your hypothesis and that, indeed, the changes you made resulted in the results you predicted and that the results aren’t by chance.
To run statistically significant tests, you need to run your test long enough to reach statistical significance. If you’ve a small sample size (i.e., if you only have a low volume of traffic to test your experiment), reaching statistical significance can take much longer.
In general, at this point, you’d need to determine 1) how long you’ll want to run your test (a week is standard for many experiments) and 2) how much traffic each version should see.
Traditionally optimizers have suggested running tests until 95% or 99% statistical significance is reached. So if you calculated that running an experiment for a week would produce statistically significant results, you’d only check the results post a week. Letting an experiment run its intended length helps optimizers rule out “chance” winners.
However, it’s also common for optimizers to “peek” at the tests’ results in the interim and choose a winner.
With peeking, a test may seem statistically significant on Monday but not on Tuesday. But as noted earlier, if you “feel” a version’s results will hold over time, it’s a bet you will make. For example, if it’s obvious soon enough that version B is “bleeding” revenue or “minting” it, you’d want to act immediately.
Peeking may not always be advisable, but there are many rules in conversion rate optimization, and there are many exceptions too.
Solutions like Adapty already do the statistical significance calculations for you, so you don’t have to be a statistician to analyze your test results:

Just try to ensure you’ve good traffic volumes to send to each version and know what a reasonable experiment length would be.
Stopping an A/B test is easy with Adapty. When your experiment has run its intended length or whenever you want to end it, click the “Stop A/B test” option and choose the version you want to make your main paywall now. Adapty will now show this paywall to all your users.
You’d also want to set targeting rules at this point.
For example, if your data has shown that users on a particular OS version convert the worst – you can handily get this data via Adapty – you might want to run a paywall A/B test only for this segment. You wouldn’t want to run the test for your entire target audience/user population.
In such cases, you need to create a segment of such users (again, this is easily possible with Adapty’s cohorts feature), and choose only this segment for the test:
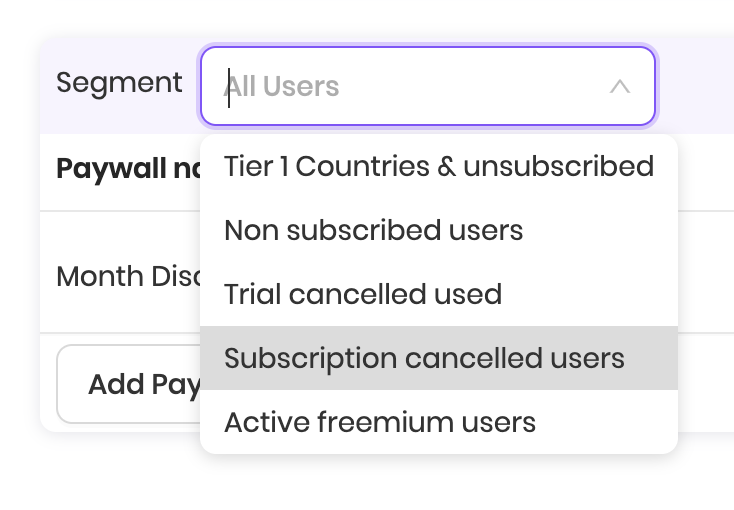
At this point, you’ll also have to define the traffic split to your different versions. For example, if you expect 2000 people to hit your paywall, you might do a 50-50 distribution: 50% of your users will see version A, and the remaining 50% will see version B. Your testing solution will randomly allocate traffic to both versions.
But if you’re unsure, you can send more traffic (say, 70%) to your current version (A) and show the experimental version (B) to just 30% of the remaining user base.
Analyzing
If you use a solution like Adapty to run your mobile app’s paywall tests, analysis gets quite easy. You get the out-of-the-box reporting on how your experiment and each version is doing:
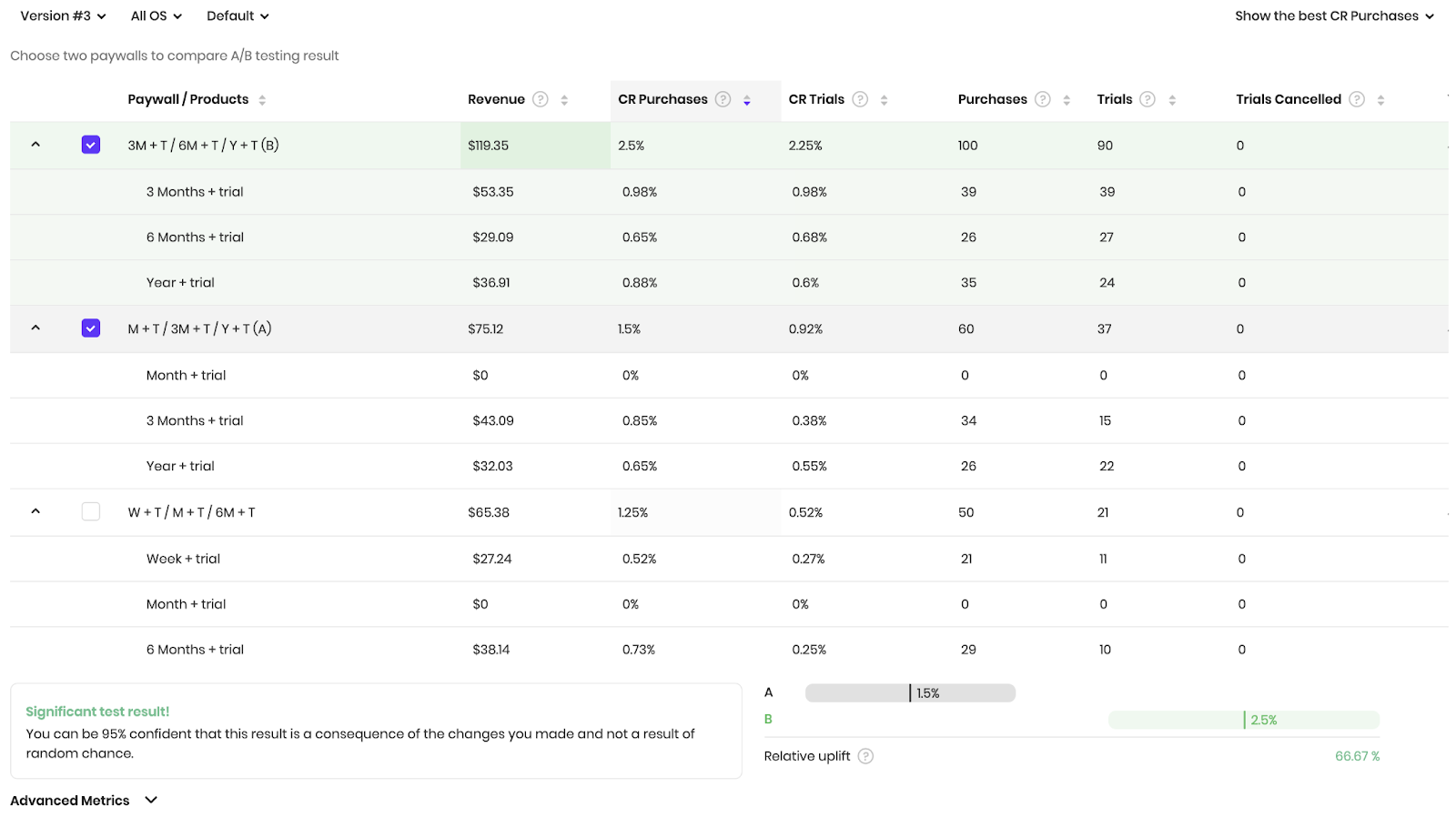
Here’s how it works:
As soon as your A/B test is live, Adapty starts collecting metrics on the performance of both versions. Adapty will tell you the conversion rate for version A and version B. Adapty will also show you the relative uplift that your experiment generates.
Note that Adapty updates an experiment’s metrics in real time.
Adapty also comes with very detailed analysis data for each version. For instance, if paywall A has three products, Adapty will start capturing the conversion metrics for each product. It will do the same for all the products in paywall B and give you a net revenue for both the paywalls and for each paywall separately. If a plan is outrightly popular with the users, such analysis can show it to you. And you can plan an experiment promoting that.
Your experiment lifecycle ends with the analysis step.
Make sure you archive all your learnings from your different experiments and use them to fuel your future experiments and guide your experimentation program.
A quick note on mobile app paywall personalizations
While we’re at it, let’s talk about paywall personalizations too.
Unlike paywall A/B tests, personalizations don’t try to find a better, more performant, or profitable version of a paywall.
Instead, personalization paywall experiments focus on making the paywalls more personalized for their target segments, so that users can connect better with them and you see more sales and revenue.
If you’re curious about how personalizations on the paywalls works, check out our post on personalized mobile app paywalls.
Wrapping it up…
When you add experimentation to your mobile app business mix, remember that it’s more of a cultural thing. Unless you embrace a culture of experimentation, you won’t fully realize it as a growth channel. Think about it: How far can a single one-off or a few ad hoc paywall A/B tests take you?
To truly unlock experimentation’s growth potential promises, you need to commit to it.
The best part is that with an app paywall A/B testing solution like Adapty, you can empower everyone on your team – even marketers with no coding skills or advanced statistical expertise – to run data-driven experiments. You can also maintain a good testing velocity as the experimentation lifecycle cuts short with Adapty’s quick test build-building capabilities. Plus, you only need development resources during the initial codifying stage, and our friendly support staff is here to help.
Check out how Adapty brings experimentation to mobile app businesses and helps them build more optimized paywalls that translate to more revenue.
Further reading:
- Paywall A/B testing guide, part 1: how to approach split-testing and avoid mistakes
- Paywall A/B testing guide, part 2: what to test on the paywall
- Paywall A/B testing guide, part 3: how to run A/B tests on paywalls and why it can be difficult
- Paywall A/B testing guide, part 4: how to run experiments in Adapty
Recommended posts