Paywall A/B testing guide: How to approach split-testing and avoid mistakes
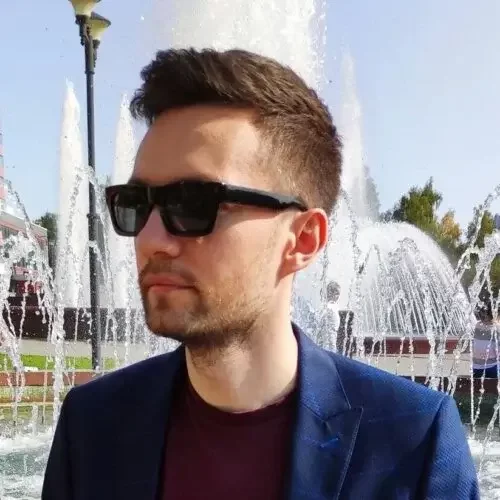
Updated: January 7, 2024
9 min read
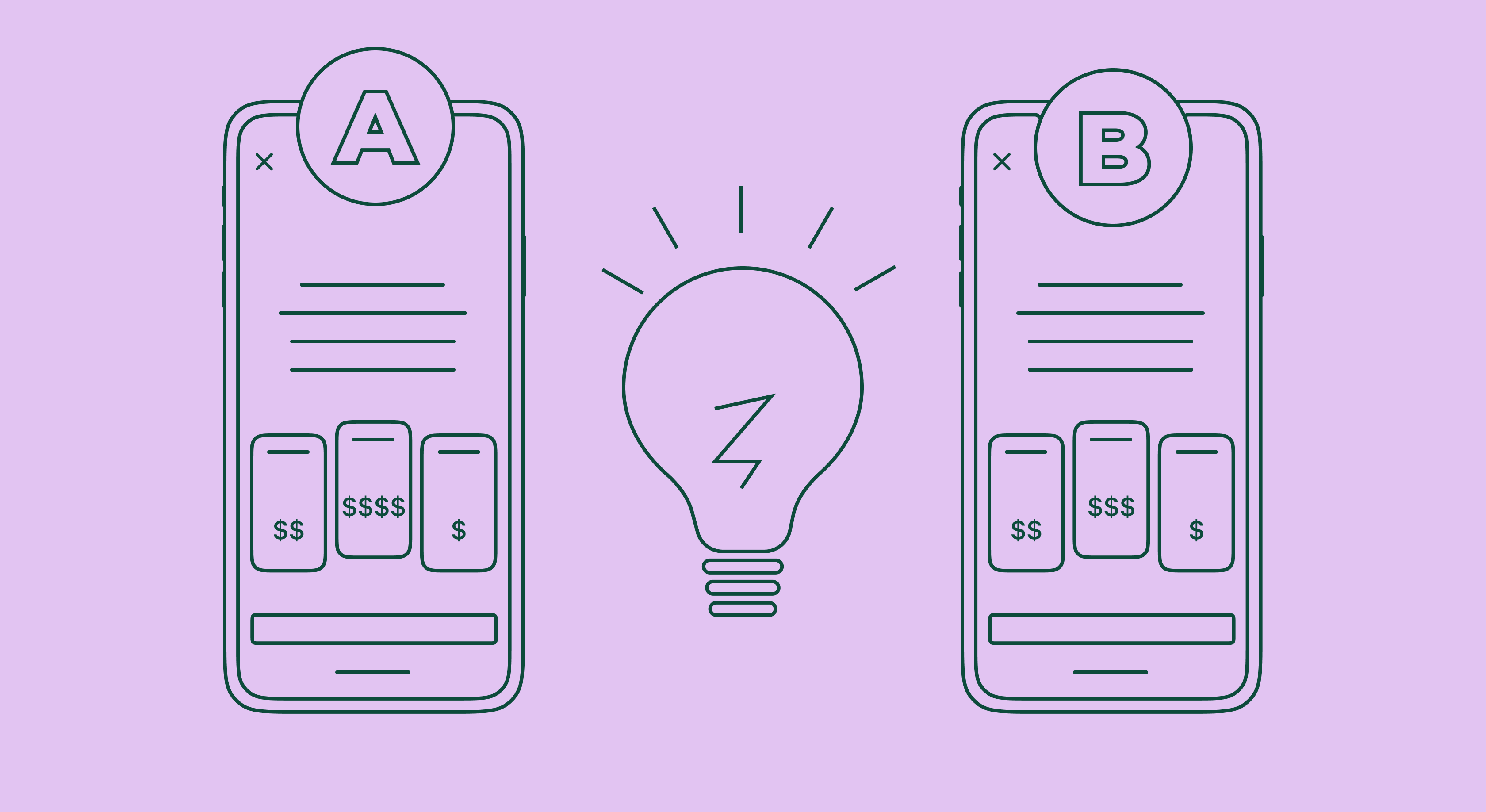
Paywalls can be designed in many different ways, have one or more pricing options, offer free trials or ask for the money right away. But the most important aspect is that it’s the place where the user should make their “life-or-death” decision – make a purchase or leave forever. That’s why it is vital to make the most out of this screen and raise the economy of your app to the highest level possible. Here’s where the A/B testing comes into play. But it’s important to know that running tests with different paywall elements may require different approaches.
This article starts our series of tutorials on paywall A/B testing, which is supposed to highlight the most important aspects of running such experiments from both marketing and technical sides. Make sure to check out the rest of the guide to get the complete overview:
- Paywall A/B testing guide, part 1: how to approach split-testing and avoid mistakes
- Paywall A/B testing guide, part 2: what to test on the paywall
- Paywall A/B testing guide, part 3: how to run A/B tests on paywalls and why it can be difficult
- Paywall A/B testing guide, part 4: how to run experiments in Adapty
A/B testing different elements require different approaches
One of the most effective tools for growing your app’s revenue is A/B testing. Let’s refresh our memory: A/B test is an experiment that is run when a paywall needs to be improved in order to be more profitable or user-friendly, but it’s hard to say right away how exactly it should be improved. Such an experiment allows you to test a certain change on your paywall within the same version of the app, without having to release a new one. Almost any element can be tested, from those directly affecting the app’s economy (pricing options, number of products, etc.) to the ones that belong to design (button shapes, colors, call to actions, etc.). However, there’s an important aspect you should pay attention to when launching an A/B test.
Would you measure the results of a test with a new button color in the same vein as a test with a different price option? On the one hand, such tests would look similar – you change one element on your paywall, test it against the original variant, and wait until you get the statistically significant result. But the thing is, the effect produced by each of these changes would be different.
When testing design elements, call to actions or any other textual information on the paywall, we can clearly see and even measure the effect of such a change right away. These elements do not directly affect the economy of your app, but all the other metrics that you’re trying to improve. Thus, having a positive stat significant result with a new button color, you can safely admit that the test was a success, people do tap the button more willingly, and this new color works, for what it’s worth. But can you apply the same approach to a test with a new subscription, for example?

All the changes that directly affect the economy of your app should be evaluated more precisely and with regards to the future revenue. It’s virtually impossible to get a stat significant result if you test a higher price for your annual subscription, as the goal of such an experiment lies in a different area. When changing a price option, for example, you should beforehand understand what you’re trying to achieve.
Placing a higher price for your subscription will surely negatively affect the bounce rate at the moment, which could be considered a failure, if you approached this as a simple A/B test. But you’re doing this experiment in order to boost your LTV and increase your annual revenue, so as long as the bounce rate is no higher than -15% – you’re safe. But it’s still early to say if the test is successful, especially if you test the price increase with your annual subscription. A good idea would be to follow the cohorts of the two paywalls involved in the testing and keep an eye on the retention rate. You may get less subscribers, but if they are willing to pay more and stay longer, thus boosting the LTV of your app, that’s a success.
2024 subscription benchmarks and insights
Get your free copy of our latest subscription report to stay ahead in 2024.
Quick tips to avoid A/B testing mistakes
A/B testing is a complex process that includes numerous components you should pay attention to, in order to make the whole thing work and get proper results. Sometimes even the slightest neglect can make your experiment invalid or even worse, bring you false results, that will become the ground for further experiments. So it wouldn’t hurt mentioning a few aspects that you should bear in mind when dealing with A/B testing.
- Make sure you’ve come up with the right hypothesis. If the users don’t tap the purchase button as much as you expect them to, it doesn’t mean you should rework the whole economics of your app right away, but maybe check if the button functions properly and the users don’t get lost in the messed up UI.
- Keep the tests simple. That means not testing more than one element and running several different tests at once. The more changes you’re trying to test, the “dirtier” results you’re going to get, as you wouldn’t know what affected what.
- Pay attention to the time periods and traffic. Make sure to dedicate enough time to test your hypothesis (usually at least 2 weeks), and check the traffic flow, it should be enough to come up with statistically significant results.
- Keep your hands away from the running test. This should become a ground rule – never change anything during the test. It’s in your interest to get the most true-to-life data, so if you found a bug or decided to change a parameter mid-test, just shut the experiment and start anew.
- Learn to interpret results and deal with analytics. Most of the A/B testing frameworks at the end of the experiment will give you a hint or even firmly state if the test was a success or a failure. But for many reasons you may want to look into the raw data, so make sure the framework you’re working with supports modern attribution and analytics systems (e.g. Google Analytics). And don’t be ashamed to let professional analysts help you – the result is all that matters.
- Don’t be afraid of failed experiments. If your A/B test shows that the original version has won, you shouldn’t approach this as a mistake, as the goal of every experiment is to get information. Maybe a higher price didn’t bring the estimated results, but at least you’ve found the highest amount your users are willing to pay.
Experimenting with different aspects of your paywall, you’d be able to easily grow your revenue and improve other metrics. The look and logic of the paywall may vary significantly, depending on the niche, audience, specific features of the app, etc. So it’s up to you to find what works best for your app. And if you wonder what to start with and which elements to test in the first place, have a look at the next chapter of our series, where we’ll tell more about structures, main elements, handy tricks, and many other useful aspects of A/B testing.