Subscription analytics: why it’s hard, and 3 strategies to improve results
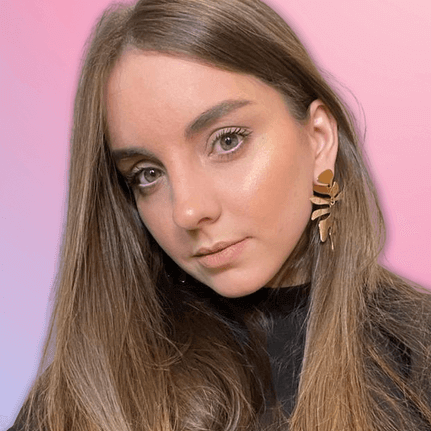
Updated: September 9, 2024
23 min read
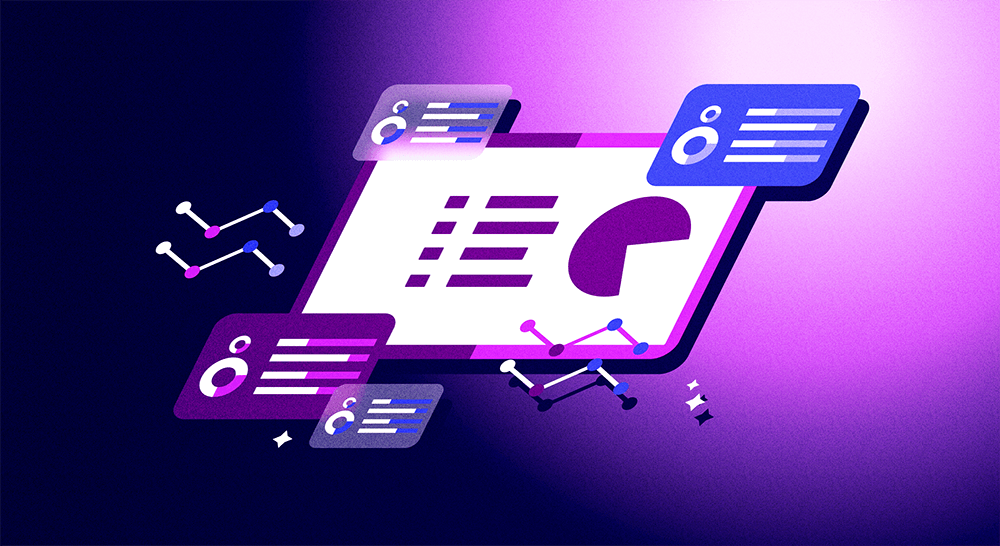
Let’s get one thing straight.
Subscription analytics is the joy and bane of every app owner in the threatening jungle of mobile apps. Nearly all developers who cracked the code of a successful subscription strategy today started just like you: stumbling upon the obstacles of comprehending the challenges of subscription metrics analytics.
With so much data to process and analyze, it can be difficult to gain meaningful insights into user behavior and subscription revenue. Moreover, factors such as consumer preferences, market competition, and data privacy regulations further complicate the subscription analytics process.
Despite these difficulties, subscription-based models are critical for the future of mobile apps, as people increasingly rely on smartphones for accessing content and services. They no longer choose laptops over smartphones – they want fast, top-notch experiences that are just a tap away from their fingers.
So, let’s recapitulate before continuing.
- Subscription-based models enable app owners to turn one-time downloads into lifelong subscribers. AKA: long-term revenue.
- Subscription apps are based on the success of understanding subscription analytics.
- Subscription analytics comes with a series of challenges that you’d learn how to overcome throughout the length of this article.
So, buckle up, grab a coffee, and prepare to conquer the wild jungle of subscription analytics!
Why is subscription analytics hard?
Simply put, subscription metrics analytics becomes a challenge for app owners because it involves constant monitoring in order to understand whether your app revenue data is positive or negative.
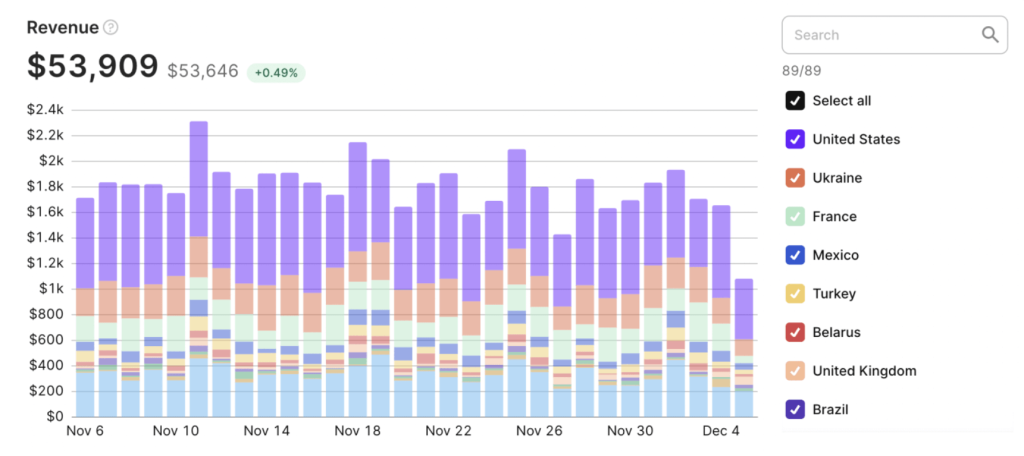
Firstly, app owners need to accurately estimate app revenue and track data to gain meaningful insights into user behavior and subscription revenue. This includes analyzing a diverse range of data sources, data formats, and data integrations, including app store revenue, payment gateways, and ad networks.
IDFA changes and SKAN attribution have significantly impacted the ability to track user data, affecting churn prediction and retention strategies. With Apple’s IDFA changes, user privacy has been prioritized, limiting the granularity of data collected, which in turn influences the accuracy of churn prediction models.
The introduction of SKAN limits attribution, further complicating user behavior analysis. As a result, marketers are increasingly relying on alternative data sources, probabilistic models, and cohort-based analyses to predict churn and develop retention strategies.
Another significant challenge is identifying the correct attribution model for a subscription-based business. Cohort segmentation issues and predicting churn can also make it difficult to track user behavior and subscriptions over time.
Privacy and data security also enter the game for subscription apps. Evolving data privacy regulations and laws can also pose a challenge to subscription metrics analytics. App owners must ensure that they comply with data privacy regulations while collecting and analyzing data in order to successfully apply subscription strategies.
Welcome to the data jungle
Subscription analytics in mobile apps can be a wild ride, with a diverse range of data sources, data formats, and the need for data integration.
Let’s start with the diversity of data sources. There are so many different platforms and channels that app owners must monitor to gain meaningful insights into user behavior and subscription revenue. This includes app stores such as Google Play and Apple App Store, payment gateways, and ad networks.
The existence of diverse data sources plays a crucial role in subscription analytics, empowering businesses to optimize their services. According to a 2020 McKinsey study, firms leveraging extensive data sources experienced a 30% increase in annual revenue. Various data sources, such as social media, web analytics, and customer behavior logs, contribute to comprehensive subscription analytics. For example, Adobe’s Digital Economy Index (2020) reported that 80% of digital subscriptions are now based on consumer data collected across multiple channels.
Next up, we have the differences in data formats. Each platform and channel has its own unique data format, which can make it challenging to compare and analyze data. Then, we have the need for data integration advertising platforms such as Facebook and Google AdWords to get a complete picture of their subscription strategy and app revenue data.
Attribution and cohort analysis
Subscription analytics relies heavily on identifying the correct attribution model and cohort analysis. Attribution models are critical in attributing revenue generated from subscriptions to specific marketing channels. App owners need accurate attribution models to allocate marketing budgets effectively.
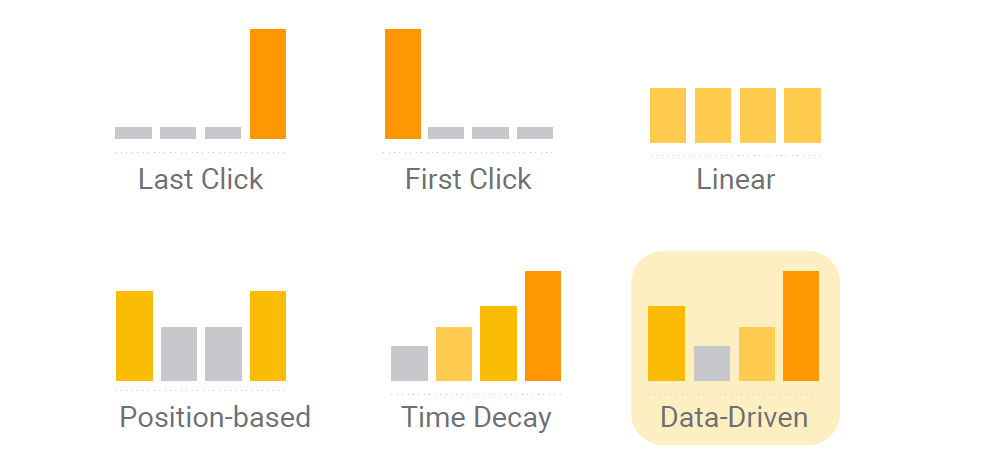
Cohort analysis involves segmenting users based on a specific characteristic to identify patterns related to user behavior. For example, it can reveal that users who sign up during summer months have a higher churn rate or users from social media channels have a higher lifetime value.
Without accurate attribution models and cohort analysis, app owners may make decisions based on incorrect data, leading to ineffective marketing campaigns and loss of app revenue.
Predicting churn and keeping users
Predicting churn and keeping users engaged is crucial for a subscription-based app. To improve retention and churn, app owners must monitor user engagement metrics and track user feedback to address concerns and identify areas for improvement that could potentially hurt the user experience.
Factors influencing churn include inadequate customer service, lack of personalization, and pricing issues. A 2021 study by Deloitte revealed that 70% of customers consider personalized experiences essential. Predictive models, such as machine learning algorithms like logistic regression and random forests, help identify churn indicators with up to 80% accuracy. Implementing retention strategies involves complex, data-driven methods, such as targeted campaigns, dynamic pricing, and customer segmentation. Companies like Amazon have reduced churn rates significantly through advanced analytics and personalized recommendations, showcasing the significance of these strategies.
Developing accurate models for predicting churn involves machine learning algorithms analyzing app usage data to identify patterns. This helps predict when a user may cancel their subscription, allowing app owners to implement retention strategies such as personalized offers, incentives, or rewards for users who engage regularly. By keeping users satisfied, app owners can increase app revenue estimates and build a loyal user base.
Privacy and data security
When collecting user data, app owners must comply with legal materials and data protection regulations such as GDPR, CCPA, or COPPA. These regulations require app owners to obtain user consent before collecting data, ensure that the data is accurate, and give users the right to access and delete their data.
To preserve user privacy, app owners must also provide clear explanations of their data collection and analytics practices. This includes detailing what data is collected, how it will be used, and who it will be shared with. App owners should also provide users with a clear opt-in and opt-out mechanism for data sharing.
To protect sensitive data, app owners should implement security measures such as encryption, secure authentication processes, and regular security audits. They should also limit access to sensitive data and ensure that all parties involved in the data processing chain comply with data protection regulations.
Strategy 1: simplifying data collection and integration
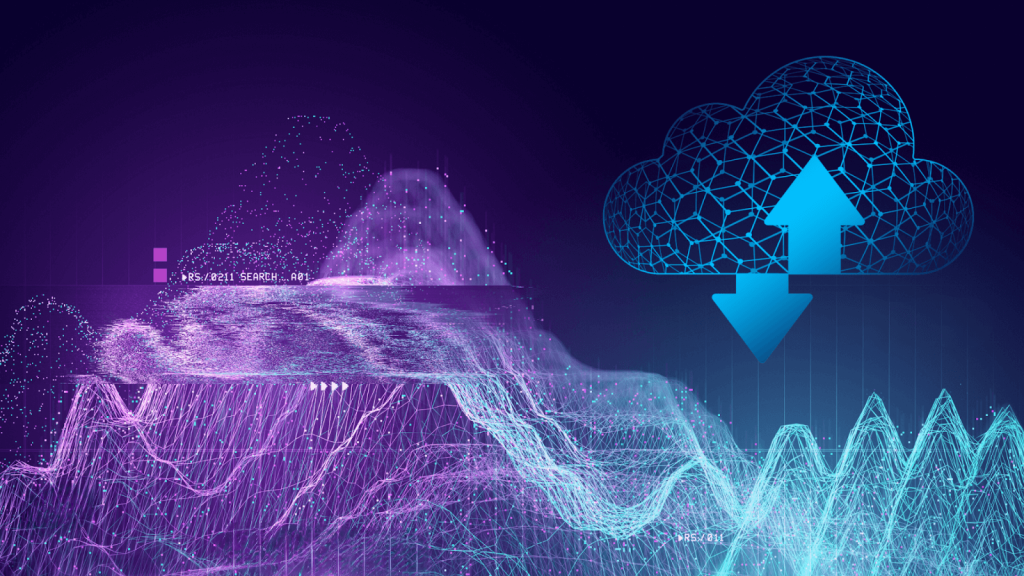
Alright, let’s talk about strategy. The first one on our list is to simplify data collection and integration. This involves choosing a common data format, creating a data warehouse or data lake, and automating data handling tasks. Sounds complicated? Fear not, we got you.
Choosing a common data format and converting data from different sources helps to ensure data consistency and accuracy. Creating a data warehouse or data lake provides a centralized location for storing and accessing data. It’s like having a one-stop shop for all your data needs. Automating data handling tasks such as extracting, transforming, and loading data can save time and reduce errors. It’s like having a personal assistant that takes care of all the tedious data work for you.
One data format to rule them all!
Choosing a common data format such as JSON or CSV and converting data from different sources simplifies data integration. It helps to ensure data consistency and accuracy, making it easier to analyze app revenue data. Just like how choosing a common language makes communication easier, choosing a common data format makes data integration a breeze.
A central home for your data
A subscription analytics data warehouse is a centralized repository designed for reporting and analysis of subscription apps’ performance. It consolidates data from various sources such as app revenue estimates, app revenue data, in-app purchases, user engagement, and subscription billing platforms, enabling better identification of patterns and trends.
In contrast, a data lake is a repository for unstructured data, suitable for multiple purposes, including machine learning, and predictive analytics. It allows the storage of raw data without predefined schemas, making it easier to access vast amounts of data and run complex analytics algorithms for tracking subscription apps and estimating app store revenue.
Whether opting for a data warehouse or data lake, having a centralized repository ensures data consistency and accuracy when working with subscription business analytics. It allows you to gain deeper insights into app performance, make informed decisions about your services, check app revenue, and ultimately increase your estimated app revenue.
The ETL magic
Automating data tasks is like having a magic wand that takes care of all your data tasks for you. Extracting, Transforming, and Loading (ETL) tools such as Talend and Apache NiFi can automate these tasks and provide an efficient way of managing data.
Extracting refers to retrieving data from various sources such as databases, APIs, or flat files. Transforming involves processing the data to clean, format, and convert it into a format that can be easily analyzed. Finally, loading involves moving the transformed data into a data warehouse or data lake where it can be accessed by business intelligence tools for analysis.
Automating these tasks saves time and reduces errors that can occur during manual data handling. With ETL tools, data can be transformed and loaded into your data warehouse without any effort on your part, allowing you to focus on making informed decisions about your subscription business.
Strategy 2: harnessing the power of advanced analytics
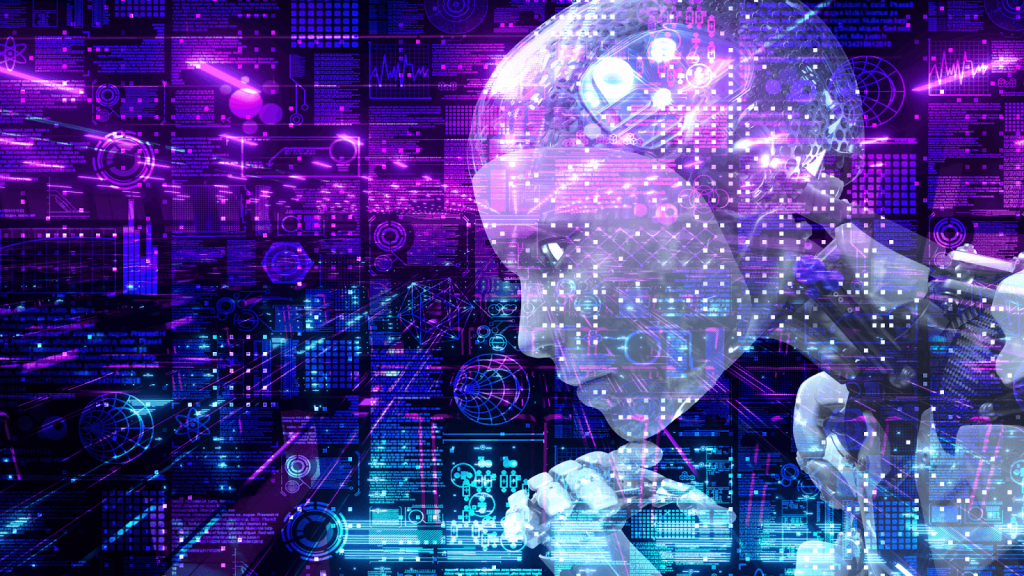
Among the critical metrics for subscription businesses, Monthly Recurring Revenue (MRR) stands out. MRR provides a more comprehensive picture of revenue trends compared to raw revenue figures. This metric considers subscriptions of different durations and is based on active subscriptions, making it a useful daily metric for analyzing trends.
For subscription apps, Customer Lifetime Value (CLV) allows you to determine the worth of a newly acquired customer and what you can invest in the relationship in order to maximize CLV while remaining profitable.
Churn rates are crucial metrics that suggest customer dissatisfaction and result in reduced customer acquisition, retention, and revenue growth.
Average Revenue per Account (ARPA) or Average Revenue per User (ARPU) measures the average customer spend on subscription plans. Strategic cross-selling and upselling can increase ARPA without compromising customer satisfaction.
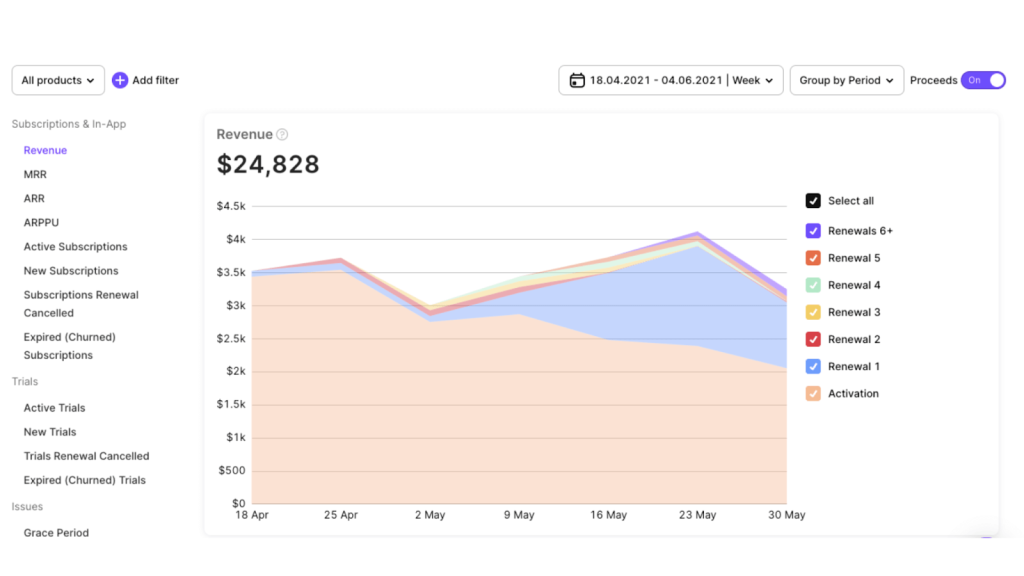
Finally, renewals are the money-maker in the subscription business model. The goal is to keep subscribers renewing for as long as possible, directly improving MRR. Revenue from renewals is reliable, even with zero new installations per month.
For a broader explanation of advanced mobile metrics to evaluate your product economy success, read our article on the Top 5 advanced mobile apps subscription metrics.
Cohort analysis and smart grouping
When choosing cohort parameters, app owners must consider their cohort analysis goals. Grouping users by marketing channel can help analyze the effectiveness of campaigns while grouping by sign-up month can track user engagement over time. Cohort size should be optimized for meaningful insights.
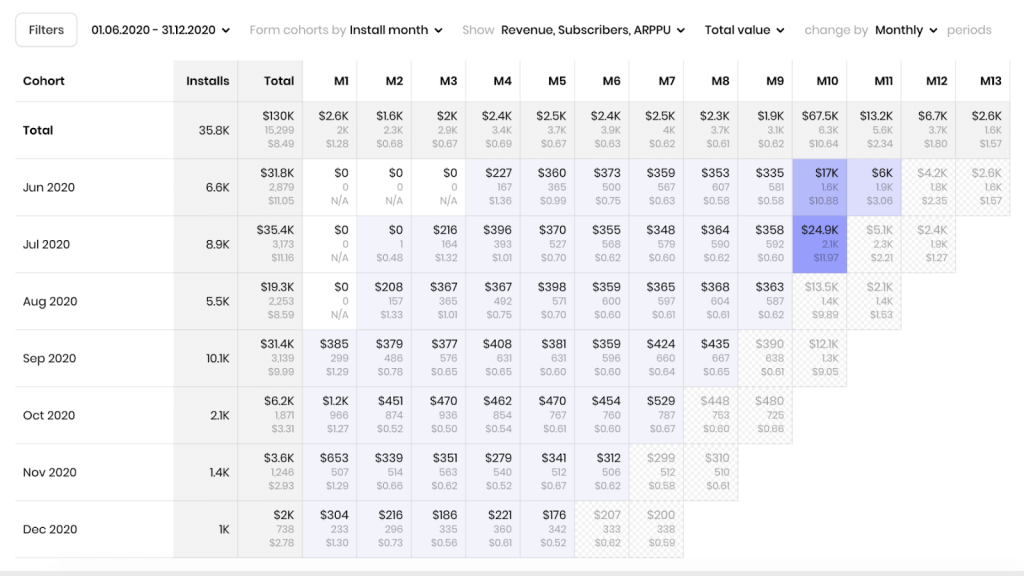
Smart grouping is another practice that groups users based on similar characteristics such as subscription plan type, user activity levels, or geographic location. Smart grouping can provide valuable insights into user behavior, such as which groups are likely to churn or generate more revenue. App owners can use this information to implement targeted retention strategies to keep users subscribed. For example, a food delivery app may group users based on order frequency or favorite cuisine to provide personalized experiences and prevent churn.
Churn and retention prediction
Churn and retention prediction involves creating machine learning models that can predict when a user is likely to churn or cancel their subscription. These models use a range of data points, such as app usage patterns, engagement metrics, and user feedback to identify patterns that may indicate a user is at risk of churning.
Once the models have been created, it’s important to fine-tune their performance to ensure accuracy. This can involve adjusting the model’s algorithms, tweaking input parameters, or using additional data sources to improve predictions.
The insights provided can be applied to marketing plans and retention strategies: app owners may use the models to identify users at risk of churn and implement targeted incentives to encourage them to stay subscribed. They may also use the models to identify high-value users who are more likely to upgrade to premium subscription tiers and tailor marketing efforts to this audience.
Real-time analytics for the win
Monitoring real-time behavior analytics is crucial to ensure a long-term relationship with your users. By tracking user behavior in real-time, app owners can quickly identify and address any issues that could potentially result in a drop in users.
For example, if a mobile app experiences a sudden increase in crashes, real-time monitoring can help app owners quickly identify the cause of the issue, such as a bug or a change in the app’s infrastructure. They can then take immediate action to fix the problem, such as releasing a patch or rolling back the change that caused the issue before it hurts user retention.
By monitoring user behavior in real time, app owners can also identify opportunities to improve the app’s functionality or usability. They can track how users are engaging with different features and make adjustments to optimize the user experience.
Strategy 3: staying on top of privacy and data security
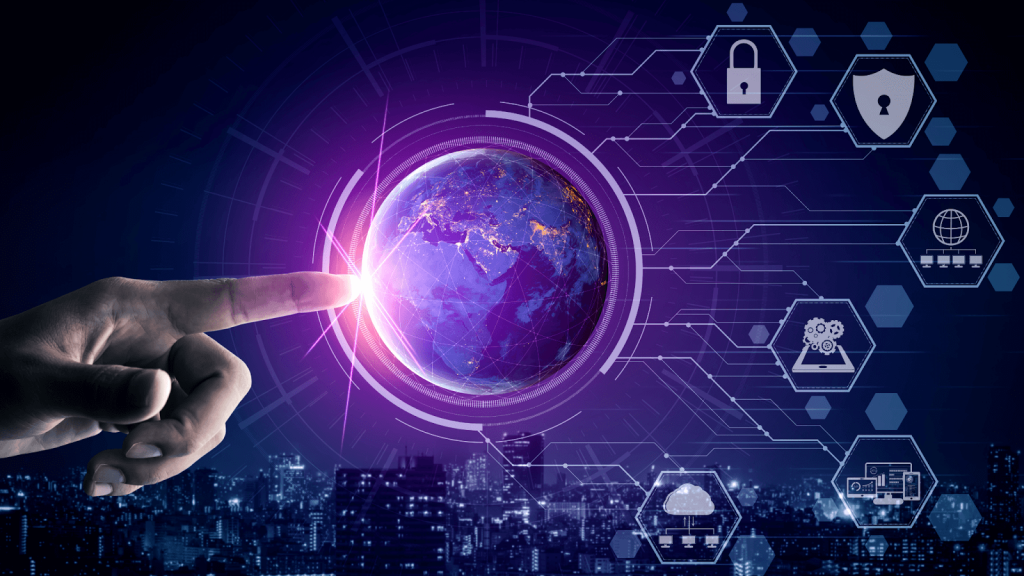
Data privacy and security are essential for app owners collecting data from mobile app users. Users entrust their personal information to the app owner, and it is their responsibility to ensure that this data is protected. Failure to do so can result in legal action, loss of trust, and damage to the app’s reputation. Additionally, data breaches can lead to the loss of sensitive data, which can have consequences for both the users and the app owner. It’s essential to implement security measures, such as encryption, access controls, and regular security audits to remain compliant with applicable laws and regulations.
Navigating data protection rules
Ensuring privacy and data security in mobile apps poses a responsibility for app owners, as they collect and store sensitive user data on a daily basis. To achieve this, app owners must be aware of relevant laws and regulations regarding data privacy and adapt to regional requirements.
For example, the General Data Protection Regulation (GDPR) is a European Union law that outlines strict guidelines for data protection and privacy. Any app that collects and processes data from EU citizens must comply with GDPR requirements or face significant fines. In the United States, the California Consumer Privacy Act (CCPA) imposes similar requirements for data protection and privacy.
In addition to complying with regional regulations, app owners must also take proactive steps to ensure data privacy and security. This can include implementing secure data storage and transfer methods, using encryption to protect user data, and regularly monitoring for security breaches.
By prioritizing privacy and data security, app owners can build trust with their users and avoid potentially costly legal and financial consequences. It is crucial for app owners to stay up to date with relevant laws and regulations and continuously adapt to evolving privacy and data security requirements.
Locking down data security
In today’s digital landscape, protecting sensitive data is crucial for any app owner. This is because sensitive information such as user login credentials, financial information, and personal details can be targeted by hackers and cybercriminals for malicious purposes.
Encrypting sensitive data is an effective method to ensure maximum data security. By transforming sensitive data into a code that can only be accessed with the correct decryption key, encryption provides an additional layer of protection against unauthorized access or theft. This means that even if the data is intercepted, it will be unreadable to anyone without the key.
However, encryption alone is not enough to guarantee data security. App owners should also regularly check and update their security systems to ensure that they are equipped to handle the latest threats. This includes implementing measures such as firewalls, intrusion detection systems, and two-factor authentication. By regularly updating their security systems, app owners can stay ahead of potential threats and prevent data breaches before they occur.
Respecting user privacy
When it comes to collecting user information through mobile apps, it is crucial to respect user privacy. This involves obtaining user consent for data collection and ensuring that any collected data is properly anonymized to protect user identities.
Obtaining user consent for data collection is an important step in respecting user privacy. This can be done through the use of clear and concise language in privacy policies and obtaining explicit consent before collecting any user data. It is important to clearly state what data is being collected, why it is being collected, and how it will be used.
In addition to obtaining consent, data anonymization is another important measure to protect user privacy. This involves removing any personally identifiable information from collected data, so that it cannot be linked back to an individual user. This is particularly important when dealing with sensitive information such as health data or financial information.
By respecting user privacy through obtaining consent and data anonymization, app owners can build trust with their users and ensure that their information is being used in a responsible and secure manner. This can lead to increased user engagement and loyalty, ultimately benefiting both the app owner and the user.
Conclusion
Let’s wrap this baby up:
Understanding your subscription analytics is a non-arguable must in any mobile app marketing strategy.
Work smart, not hard.
- Simplify your data collection and integration. Choose a common data format, create a centralized data warehouse, and automate data handling tasks through ETL tools.
- Prioritize data privacy and security by implementing robust measures such as encryption, access controls, and regular security audits to protect user data and remain compliant with applicable laws and regulations. We know. It sounds tedious and boring, but it will help you obtain consent data and build trust with users while also preventing legal and financial consequences.
- And lastly, track your metrics. Don’t just stay with the commonly tracked app metrics. Pay special attention to analytics such as MRR, CLV, Churn Rates, Renewals, and ARPA/ARPU to ensure a deeper understanding of your app’s performance. Try different segments for cohorts and smart grouping to better understand your users’ behavior over time and effectively allocate your budget for targeted offers or incentives.
These strategies are a head start to level up your app revenue. Combined with Adapty’s multifunctional features, you’ll be able to implement them with advanced analytics, A/B testing, push notifications, and in-app messaging to increase subscription conversion rates, reduce churn, and improve overall app revenue estimates.
I won’t say it twice! Work smart – not hard.
FAQs
Recommended posts