First steps to better monetization
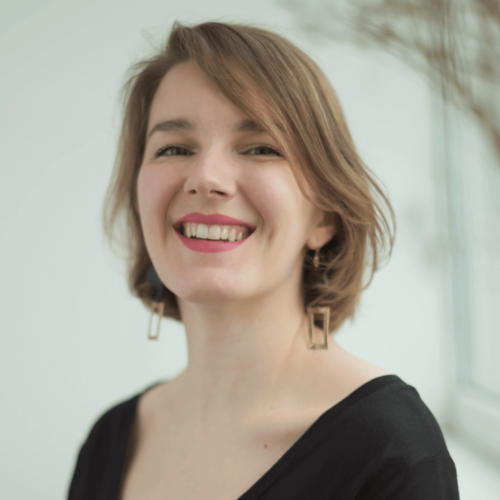
Updated: February 15, 2023
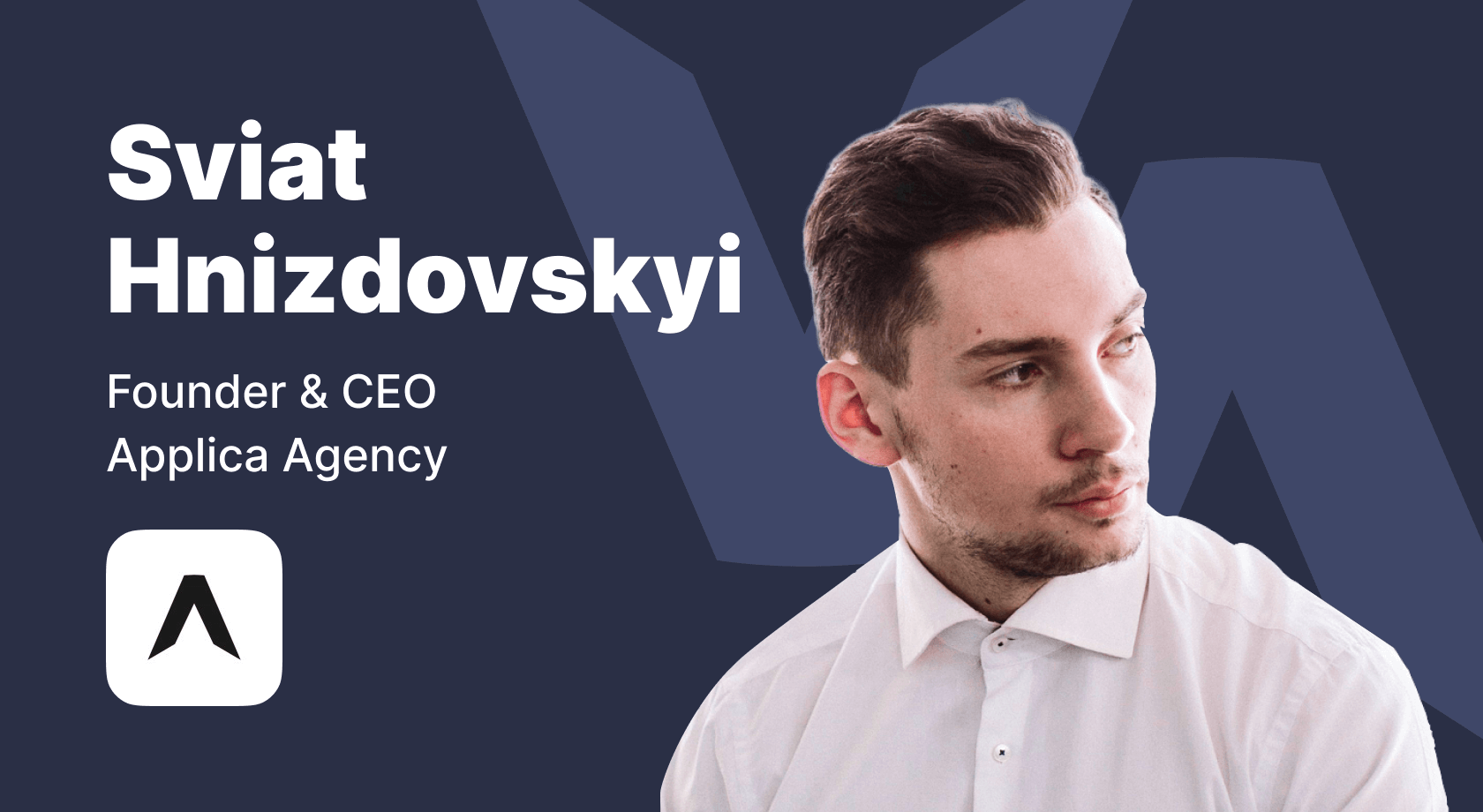
Listen to the episode
After studying social sciences at Harvard University, Sviat worked for and consulted on user activation and monetization some of the most successful apps (BetterMe, Fabulous, Drops). Now he leads his own agency Applica which helps apps monetize better.
In this episode, we talked to Sviat about how to build a monetization framework for an app and use experiments to get traction.
Sviat’s background
Sviat: basically, what I’m doing is helping mobile apps grow their revenue by optimizing subscriptions and their monetization in diverse. It’s a little bit of magic, but I would say it’s like 90% of an incremental approach.
My background was, when I started I actually was in academia and I studied Behavioral Economics and Cognitive Psychology. That was helpful for me to actually get this theoretical background on how we make decisions as human beings, how we make economic decisions, how price can influence our decisions.
After that I joined BetterMe, one of the biggest Health&Fitness apps. I started there as a Performance Marketing Manager, so was responsible for paid user acquisition. Then, at some point I got promoted to Product Marketing Manager and then eventually to Growth person who was responsible for monetization.
After that I started consulting different mobile apps. Usually those were within Health&Fitness industry, but also from Education, Entertainment on different scales. The sole purpose of that was to actually help those apps increase the lifetime value of their subscriptions, of their products.
Right now, all this consulting experience led me to creation of the mobile agency that is focusing on subscription optimization. I called it Aplica Agency. We have a very, very bright clients. Basically, that’s all the same story, helping to monetize better with the subscriptions.
Psychology for hypothesis testing
The story is, theory, of course, doesn’t translate 100% to practice. I think initially at the beginning of my career I was highly biased by theory. Like I was trying to generate hypothesis just from textbooks, but then eventually I found the golden middle. There was real feedback from users, from experiments, and of course the scope of possible hypothesis to test within, whatever, like monetization or even pricing optimization, if you take it more narrowly is enormous.
Then, I returned back to cognitive psychology and behavioral economics to actually reduce the scope of hypothesis. Well, I can’t test anything, so what’s the best thing I can do now? Maybe I’ll just take a famous cognitive bias and try to make use of it on a specific payroll. That was kind of approach I came up with and, yes, I think it’s becoming more helpful with the applied knowledge from practice I get.
Monetization framework
At some point I just started what I call now a fully operating monetization framework. At those days, we have multiple areas of optimization. Let’s say, we had an onboarding survey. Like 20, 25 questions, the famous ones almost any Health&Fitness app uses at the beginning.
We have paywalls, we have push notifications, emails. Let’s try to structure all that and see where we can still find low-hanging fruits. Maybe there are some areas we haven’t really experimented with before. I opened the spreadsheet and started mapping all the previous experiments we had, like how many experiments we had within, let’s say paywall price optimization.
Okay, we had seven experiments. On average it gave us, let’s say plus 5% ARPU increase. When doing that exercise I noticed that, let’s say, we never tested call-to-action button text on the paywall. I know for some apps it was pretty successful. I saw like, “Well, there is no experiment on that. Instead of running experiment number six on pricing that has already diminished returns because we already tested so many times, so probably we are hitting a plate of optimization. Why I don’t just look at the new area?” Like, let’s say this call-to-action text on the paywall button.
That framework helped me to identify categories within monetization optimization. There are maybe 20 or 25 different categories of what can be tested and then approach each one and see how much of an impact it has. Does it really move the needle within ARPU increase and then moving from the highest priority to the lowest.
At the end of the day, when we have the results, you can clearly say that, “Okay, let’s say you probably shouldn’t try experimenting that much with paywall design because you already did 25 experiments. You never know actually, well, that you are just doing the same thing. We can see that after experiment number 15, you didn’t reach any improvement, so let’s maybe turn our attention to something else.”
A/B tests
Vitaly Davydov: Yes. If you’re talking about A/B testing, result evaluation, in mobile apps, you have tons of metrics. Including conversion to free trial, conversion to purchase, RPPU and tons of other metrics. How did you measure performance of a particular test? How do you consider it to be successful versus fail test?
Sviat: When it comes to just solely conversion rate increase, it’s pretty simple. You have just two conversion numbers, you just open whatever, A/B Test Calculator measures statistical significances and bump, you have results.
For something like LTV optimization where you measure just two LTV numbers one by one, it’s a little bit harder. I think what is thoughted as a mistake to some degree or inconsistency for many apps, is that people ignore some of the very crucial parameters in that equation of LTV measurement.
That’s namely, refund rate, subscription retention rate, and for some, like if you’re talking about lab, subscription is also charged by rate. That’s very important and it differs a lot. Imagine you have $100 yearly subscription with 7 day trial, I would expect for some apps to have even more than like 15 or 20% of refunds for some of the apps. Of course, if you have shorter duration and smaller price, the refund rate will be of course slower. It differs between platforms, of course, between different geos demographic factors. In order to, I think the best LTV calculation, if any team would like to approach that with statistical rigors, then they should probably take a look at that as well. What is the refund rate for this particular subscription? I think that’s where the tools like Adapty can be handy for that, for sure. It’s usually neglected and there might be an assumption that it’s similar across all the subscription or the paywalls, but it has tremendous effect on user economics in total.
2024 subscription benchmarks and insights
Get your free copy of our latest subscription report to stay ahead in 2024.
Test for early stage start-ups
For early-stage startups, of course, you don’t have big sample sizes, you don’t have lots of traffic, but you still want to optimize your subscription and LTV.
I think the good idea here would be actually to start with something I call macro-level experimentation. What’s that? It’s basically, you want to identify your initial pricing, initial paywall you go with. To do that, I think you just go take top five, let’s say competitors that have good revenue, bad product.
Here’s the catch, even though they have bad product, maybe not really polished one with bad retention rates, but if they are still high on the revenue side and you can check it, I don’t know, some sort of a value or whatever. They’re probably doing something good about monetization. Instead of maybe running A/B test, your question was for how long can you wait? For early stage startups, like this A/B test, might take very long time.
Maybe just, what you can do is try this one of the best paywalls from your competitors that has particularly not the best product, but it’s still strong on the monetization side. If you’re talking more about mature companies and products, I think there is another problem, I won’t tell you right now, okay. Just, of course, there should be a meaningful period, at least a cycle, so it can’t be less than a week, I think, due to weekly seasonality, of course.
Like for some apps, especially within Health&Fitness and Education, Monday and Tuesday are usually the best days. People start new life from Monday. Imagine if you start running A/B test on Saturday and finish it on Wednesday, and the next experiment is from just Wednesday to Sunday, you are really biased because you didn’t have these two best performing days.
If you make any historical comparison, it just doesn’t make sense. That’s why it should be at least one week or even better, maybe two weeks period. The problem is, even if you gather the results within a week or two week period, what if it’s a new subscription duration and you don’t feel you know your subscription retention rates? To calculate your LTV, you have to make an assumption about that. Right?
Of course, you can go and check benchmarks and know that your multiplier is 1.5 or whatever, like what are the subscription, but it’s usually bullshit. What can be done is actually you take this first two weeks and see how many subscription cancellations you have, like what’s the percentage of that? Then, you plot a logarithmic chart, making an assumption that your curve of unsubscribed rate will have a logarithmic shape.
That’s the simplest method. It might require some mathematical, of course, skilsl, but it’s pretty straightforward. You can just do it with a piece of paper and a pen [chuckles] in the worst-case scenario, or with the help of data analyst in 30 minutes. Even if you measure and get the results in a week or two, it might take a longer period to be 100% sure for what’s your actual refund rates on subscribed rate.
I found it that some conservative companies, conservative apps, they want to be 100% sure whether this test goes with the better results or not. That they would wait maybe for a month or more to get statistically significant date on their unsubscribe rates, and I think that’s good actually. If you want to spot huge change, maybe like 20% change, at least to your LTV, of course, can be riskier. For more optimized products that have already a long history of experiments behind, that might be the case that you will actually slow down significantly with new experiments.
A/B tests what to start with
Usually when you look at a new app, founders are playing a cheap game. They would just benefit enormously by increasing the price of subscriptions by 20%, 30%, at least. Almost everyone who just has launched an app has this imposter syndrome, like, “Oh, my app isn’t that good yet. We just started early on, the product isn’t good, so I wouldn’t dare charging a high price for my subscription. I would just go with $10 per year or $20 per year.” You can see that pretty often.
The problem with that approach that you can hardly reach any profitability. You compete with the apps with the way higher price for the same subscription period. The logic here that I saw that price and elasticity worked in the way that usually the higher price, the better it is for LTV.
Of course, it has its ceiling, if you’re asked for $100 for the product that it doesn’t make sense, you will see of course high cancellation rates later on and refund rates and negative store reviews. If there is any early-stage app founder, I would suggest to actually try go and increase the price by 25%, and see how it impacts your user economics. That’s one of the ways. That’s actually what I usually start with the price and elasticity testing.
Second thing I would say is trying shorter subscription periods. Let’s say, there are some classic general like, “Let’s have a yearly subscription or let’s a monthly subscription.” By looking at the recent benchmark reports, even the one you did and I remember you shared that with me, actually shorter subscription durations like weekly or quarterly subscriptions are really promising.
Especially because if you have free trials, the conversion from install to trial is usually slightly better for this shorter subscriptions. Also, it’s because you have more frequent subscription billing periods. Of course, on the scale of the year, you will have already four billing periods for quarterly subscriptions. You can actually charge almost the same price for a quarterly subscription as you charge for yearly. I mean, of course, it depends on the product, but it can be justified actually.
Well, because quarter is a meaningful period for a lot of people to give it a try. Like if it doesn’t work for me in three months, it wouldn’t probably work for me at all. If I stick to a product within these three months, well, I’m ready to actually get charged every three months if I see the value, so what’s the problem? I think that’s once, again, if there is a yearly subscription by trying to experiment with quarterly subscription or maybe half-year subscriptions, that can be a very powerful tactic for overall ARPU growth.
Monetization at the very beginning of the user flow
Still people who pay they would pay anyways and if you don’t make enough efforts to persuade them to become pain subscribers at the beginning, you just lost them later on. It’s better to use this momentum of emotion when the user has this struggle. They see the ads, they go and experience your product like onboarding session and try to convert them right ahead, instead of waiting a few days because then, interest just falls and maybe the problem isn’t that painful anymore for the users. They are becoming more rational in their decision. Again, I think the sooner you introduce your subscription with a premium product and justify why it’s worth actually upgrading the bad ideas to your use economics.
What to try in your a/b tests
Sviat: All right. I think I have that approach with trying to find low-hanging fruits. Actually, there are things that can be done within a week or two and we can already see some improvements. Usually, I already mentioned a few of them, but we can try to structure them in a list. Once again, probably I would start with increasing the price by 20%, 30% then introducing maybe slightly shorter subscription periods in addition to what they have, then also introducing this sequential discount drop.
Like after the first initial payroll experience, the next payroll should introduce you with a lower price or better offer, like maybe try extended trial period or something else. Those are the areas you can find almost within any app that’s under-optimized, so it’s easy to jump there and make some changes.
One more thing I would really look at is paywall design layouts. I think there are now two clear patterns you can see across many apps. The first one is where you have a single price, and you have either explanation how the trial period works, like Blinkist case we will already covered, or you have some bullet points with core value proposition about the application. Like, “Here are three features or three benefits you get by upgrading to premium subscription.” That’s one pattern you can clearly see.
The second pattern is emphasizing more, not on the product benefits, but on the subscription price. What I see is usually apps introduce three pricing options. Usually have a short subscription period, like weekly or monthly subscription. Then, you have something like usually yearly or half-year or quarter subscription in the middle, which is usually the best ARPU subscription.
You want to actually make it default subscription, highlight it somehow with the design, UI patterns. The third one is usually something used for Decoy Effect like the famous economist paywall, if you’ll remember where they had like digital version of economist for $39 than print version for $100 and print plus digital version for $100. The two options didn’t differ much, but you just don’t really take into account that $30 bucks option and like psychological effect, you just compare the two last options and there is a clear winner.
It’s easy to make choice, so it’s called Decoy Effect. I see many apps use that as well, especially with lifetime. The Decoy Effect can work in two ways. The first one, you can have two very similar prices. Let’s say you have a yearly subscription for $50 and lifetime for $55. It’s very clear that lifetime is very better and the price difference is negligible. You can use that actually to increase conversion rate to your lifetime subscription, for example.
The second tactic that can be used here is to have two very different prices. It’s what Calm, for example, uses for their lifetime subscription. It runs for something from $300 to $400 sometimes, depending on whether you can find a discount for that or not. The price looks enormous, like $400 for a lifetime subscription for a meditation app.
That creates a very strong anchoring effect. Like, “Okay, if it’s so expansive, it’s probably really valuable.” There is some value to that $400. I would just go for a yearly subscription for $70 or $80 and get almost the same value, and the user can have this thought processing in their mind when making that decision. If I see the value, I wouldn’t probably use the app for five years, so I’m still better of just giving you the try for one year. I have really better offer here. I it’s like of six times longer price compared to a lifetime. Lifetime is just used as an anchor to create this value perception of really high value of the product, yes.
Further reading