Why predictive analytics is important for mobile app growth
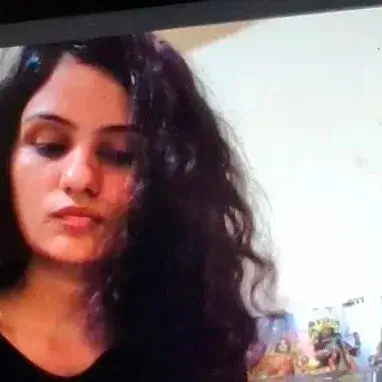
September 9, 2024
13 min read
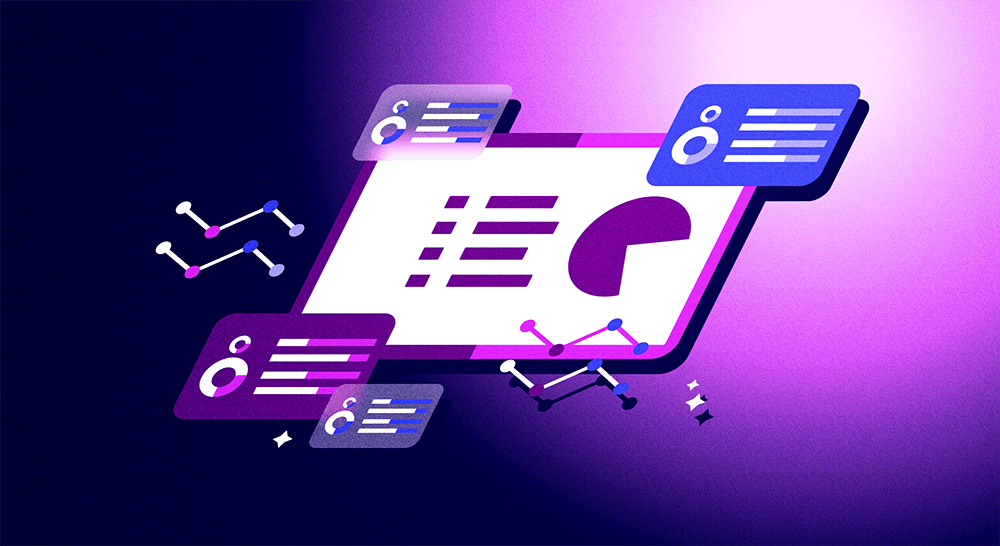
Mobile app makers who use predictive analytics compare it to having “a crystal ball for future growth.” And rightly so. If analytics tells you what’s happening in your mobile app business right now, predictive analytics shows you what’s likely to happen in the future.
And by offering insights into the future, predictive analytics empowers you to make more proactive decisions today. For example, if you’ve got a subscription app, and if predictive analytics signals a cash flow issue in the next six months, you can try to redistribute your current user base (by pushing a specific subscription plan). This way, you can better navigate or even avoid potential financial challenges before they arise.
So, let’s see how predictive analytics actually works and how it helps you make better decisions today by offering a sneak peek into your app growth’s upcoming challenges and opportunities.
What is predictive data analytics?copy link
Simply put, predictive data analytics uses historical data to predict the likelihood of future events. For mobile apps, this translates to:
- Using past user behavior data to predict future user behavior.
- Using past in-app sales data to predict future sales.
- Using past cash flow data to predict future cash flow.
And so on.
But how does predictive analytics actually do all this? Let’s understand how predictive analytics works before seeing how it applies to mobile apps.
How predictive data analytics workscopy link
Predictive analytics applies complex algorithms, machine learning, statistical modeling, AI, and more to your historical data and analyzes its patterns to generate predictions about future outcomes.
The best way to understand how predictive analytics works is to see where it fits within the business intelligence spectrum. See the graphic below for reference:
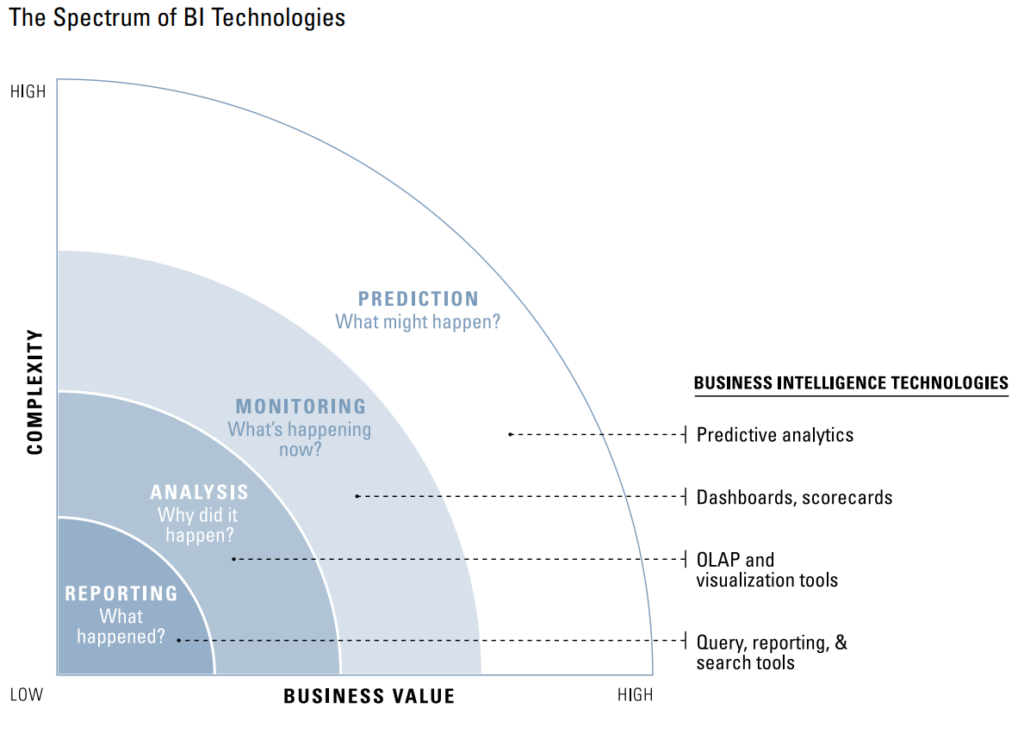
As you can see, on one end, you’re looking at what has happened in the past or is currently happening in your business. For instance, for your mobile app, this data is your past user, sales, or revenue data.
And, on the other end, you’ve got predictive analytics showing you what might happen in the future, essentially telling you what your user base, sales, and cash flow might look like in the future. (By the way, the part where you analyze what’s happened so far is descriptive analytics.)
Also, once you know what’s most likely to happen, you enter into prescriptive analytics, meaning that you start thinking about what you can or should do NOW based on things you foresee. Here’s how this works:
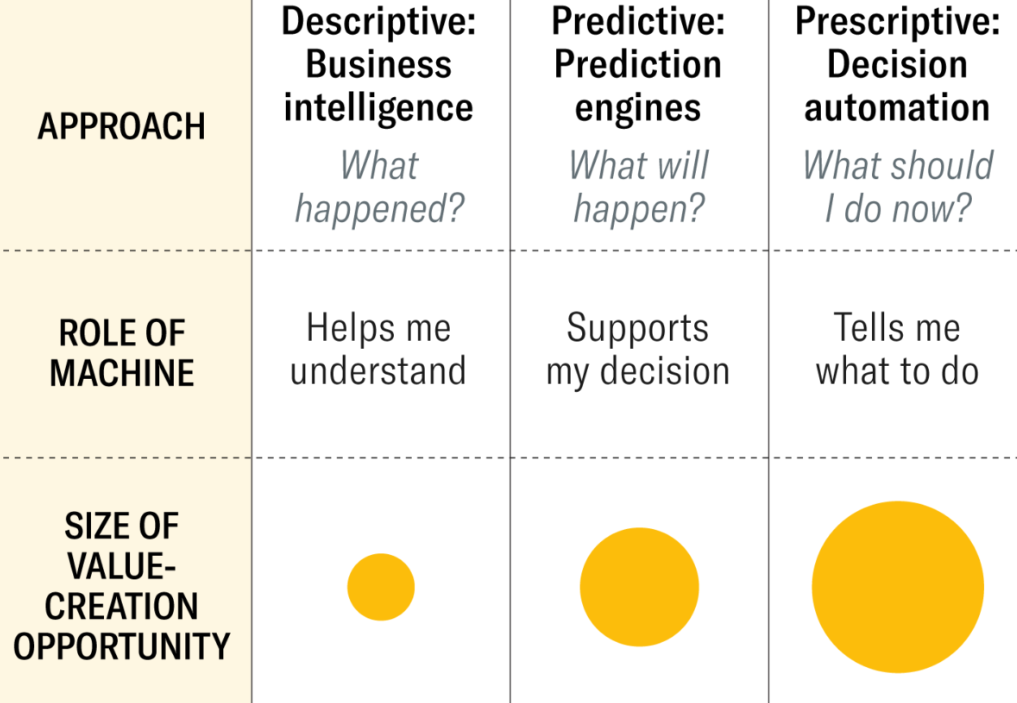
Let’s now see how all of this comes together for mobile apps.
How does predictive analytics work for mobile appscopy link
Applying predictive analytics to user data
Applying predictive analytics to your user data can help you identify your most profitable user segments.
It can also help you spot the users who are likely to have the highest lifetime value (LTV).
Predictive analytics can also pinpoint users who are at a high risk of churning.
As you can imagine, these insights on your user segments can help you work on your retention strategies. For example, if you see that your weekly subscribers are the most valuable, you can plan feature releases using a weekly cadence. This way, your weekly subscribers will consistently experience the value your app provides… and stay.
Applying predictive analytics to in-app sales data
Applying predictive analytics to your in-app sales data allows you to forecast sales for all your in-app products.
It also helps you identify the products that will be the most profitable in the long run.
Additionally, predictive analytics can highlight products that may perform poorly, allowing you to address potential issues early.
For example, when you learn how your products will likely do, you can hypothesize ways to maximize their sales. For example, for products that aren’t doing well or won’t likely do as well as you would have liked, you can try A/B testing their pricing to see if it makes a difference.
Applying predictive analytics to revenue data
Applying predictive analytics to revenue data helps you predict your app’s cash flow.
If your revenue data foretells cash flow issues on the horizon and you know which products can bring the most revenue in the short term—both these insights can be drawn with predictive analytics—you can run targeted offers to boost their sales and generate some quick capital.
Likewise, you can plan for your app’s long-term financial health using predictive analytics and finetuning your current sales and marketing efforts.
Now, before we see how you can add predictive analytics to your mobile app, let’s quickly look at some models that power predictive analytics.
Types of predictive analytics modelscopy link
There are many types of predictive analytics models. The one you choose for your forecasting depends on 1) the nature of the data you’re analyzing, 2) the kind of business problem you’re trying to solve, and 3) the type of insights you’re trying to draw. Let’s explore how some of the most popular predictive analytics models work.
Time series models
Time series models are designed to identify patterns like seasonality, trends, and cyclic behaviors in data collected at consistent time intervals. For example, if you have a subscription mobile app with revenue data collected weekly, biweekly, and monthly, you can use time series models to uncover all the patterns that exist in it to predict your future weekly, biweekly, or monthly revenue predictions. In this case, your subscription lenghts are the “time series.”
Because seasonality, trends, and cyclic behaviors are standard features in revenue data—especially for businesses using the subscription model—time series models are commonly used in revenue forecasting.
At Adapty, we use gradient boosting for our predictive analytics for subscription apps. Gradient boosting is a popular time series modeling technique.
While we’re talking about time series models, let’s discuss forecast models too. Forecast models are predictive models that can use various data types to forecast future values.
Unlike the time series model (where the data is collected across consistent time intervals), the forecast model can offer predictions even when it’s fed data that’s not time-dependent.
So, if you want predictions about in-app purchases that are different from subscriptions, the forecast model can help you. Also, the forecast model is like an ensemble model that may use multiple prediction models and techniques!
Classification models
Classification models analyze your data for any classes that exist in it.
For instance, your mobile app can have different categories of users—such as buyers, loyal customers, active users, and those likely to churn, to name a few. With classification models, you can understand the data points that identify these classes and then use them to predict which class a particular user will belong to.
With classification predictive modeling, you can predict if a user classifies themselves as a buyer, and then use targeted marketing to convert them or get them to make repeat in-app purchases.
Regression models
Regression models analyze how single or multiple data points impact target metrics.
For example, using linear regression, you can explore how data like the number of app sessions per week, the average session duration, and in-app interactions tie to retention and then predict user retention based on historical data.
This isn’t an exhaustive list of predictive models, but they’ll give you a fair idea of how predictive analytics works for mobile apps. With that, let’s finally see how you can add predictive analytics to your mobile app!
How to add predictive analytics to your app copy link
The best way to add predictive analytics to your mobile app, especially for growing your revenue, is to use a revenue platform like Adapty that comes with built-in predictive analytics. Note, however, that as of now, Adapty only offers predictive analytics for apps using the subscription model.
A peek into Adapty’s predictive analytics model
At Adapty, we power millions of in-app transactions for subscription apps, which means we have access to a lot of transactional data. This anonymized data is used to train our predictive analytics algorithms.
In addition to using our general transactional data, Adapty’s predictive model learns from your app’s transactional data as soon as you add Adapty to your app. This ensures even more accurate predictions.
Adapty’s prediction model uses gradient boosting, a highly accurate machine-learning algorithm designed to work with large datasets.
Check out our documentation for more on how we’ve designed our predictive analytics model.
Things Adapty’s predictive analytics can “predict” for your subscription app
Predicting your subscribers’ lifetime value.
One of the most popular use cases for Adapty’s predictive analytics is predicting the lifetime value that your different user cohorts will potentially bring:
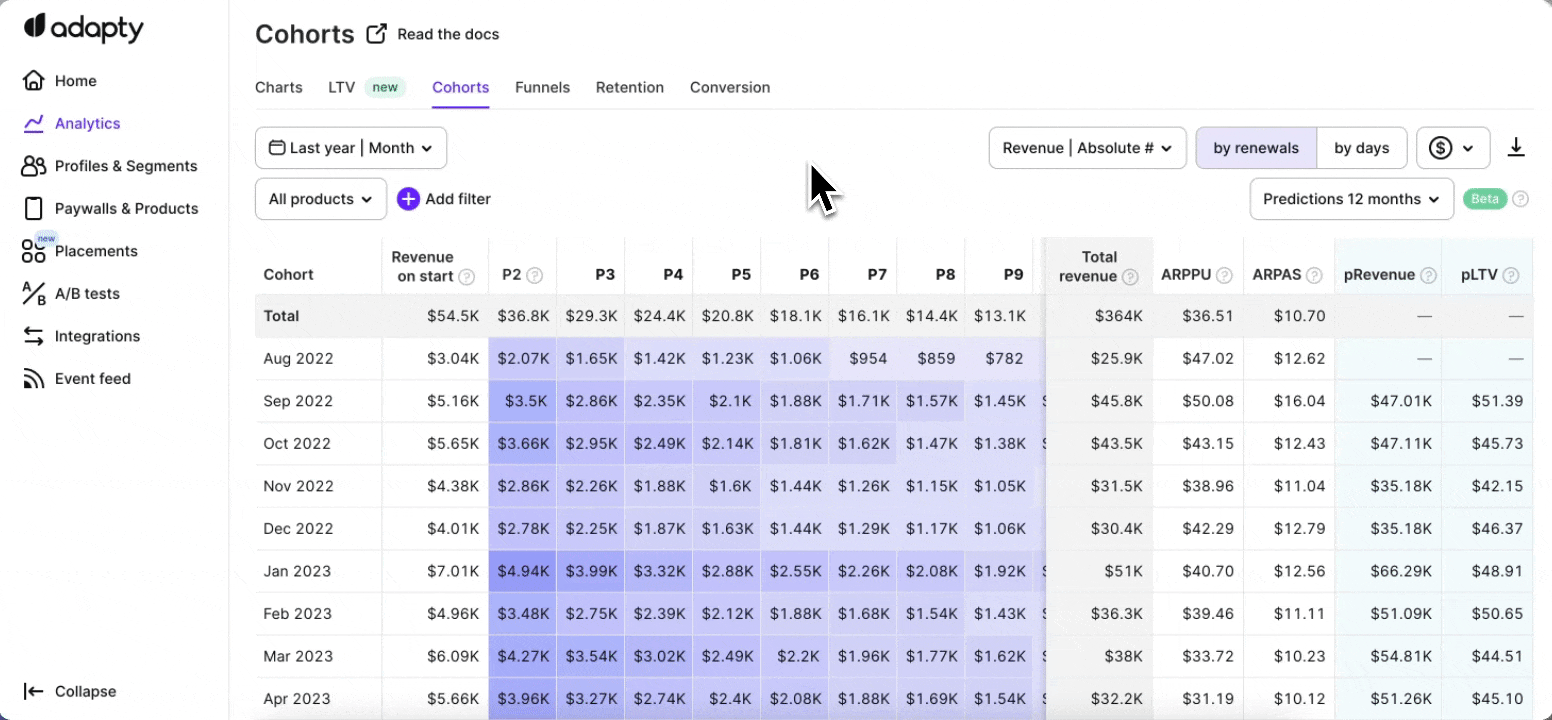
Predicting your app subscribers’ general buying behavior.
With Adapty’s predictive analytics, you can also learn how your cohorts (or user segments) will likely spend in the future. This makes spotting your most profitable segments easy, so you can finetune your marketing to them.
Predicting your products’ performance.
Adapty also comes with powerful filtering capabilities that you can use to find out the products that will be likely driving your predicted LTV data. This can help you optimize your sales and marketing efforts across your different products:
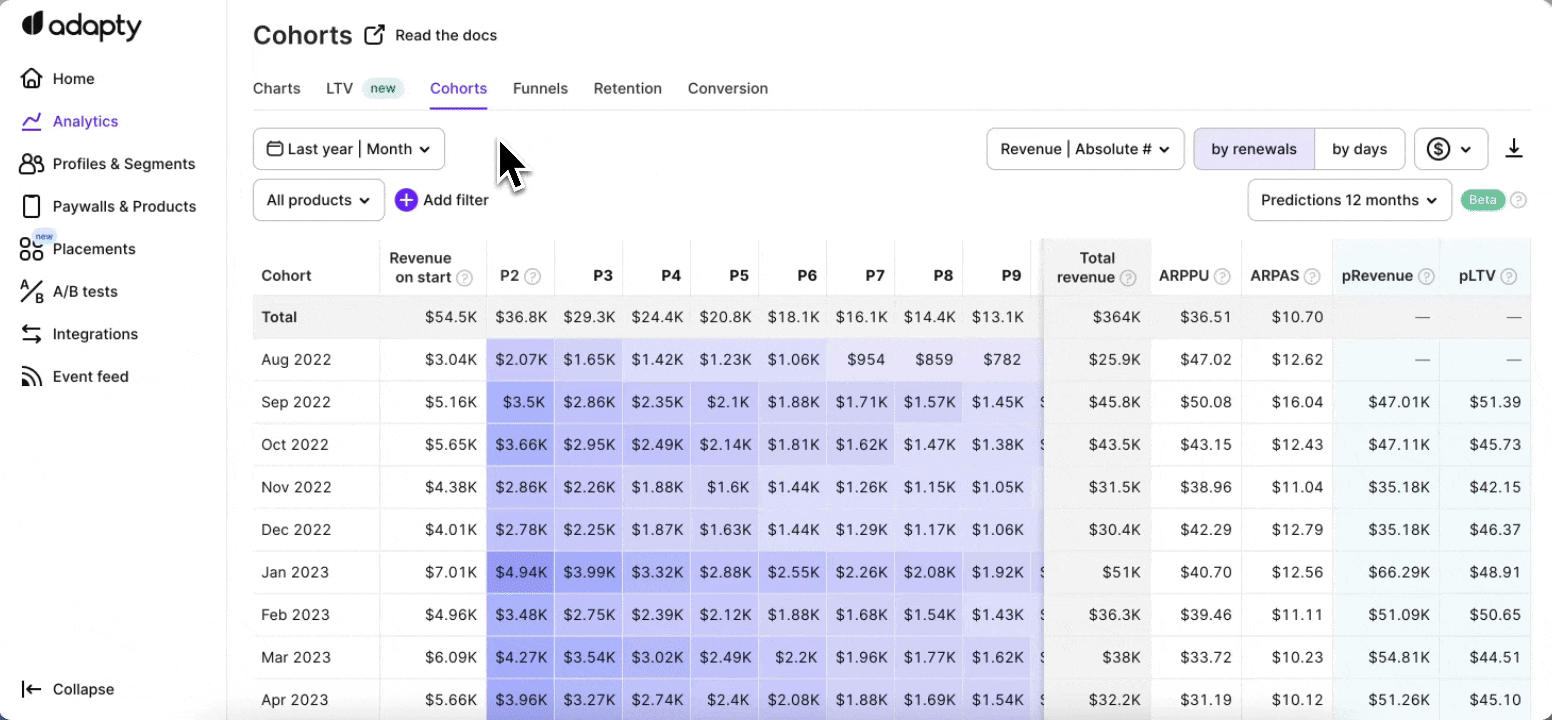
Overall, with Adapty’s predictive analytics, you can get a good sense of what your revenue will look like for up to a year into the future.
While predictive analytics can help you grow your mobile app revenue, it’s not without limitations.
The one caveat when using predictive analyticscopy link
There will always be things that predictive analytics can’t factor in—competition, for example. So, while predictive analytics can help you make more informed decisions in the present, it can’t account for any external factors that can also impact outcomes.
So, instead of relying only on predictive analytics to estimate what the next 12 months could look like for your app, use a more holistic view of your market.
It’s only when you combine predictive analytics with factors like market research, user feedback, and competitor analytics that you can clearly see what lies ahead for your app.
Also, data quality is the key. The better data that predictive algorithms get, the more accurate their predictions can be.
Wrapping it up…copy link
If your app has been around for some time and you have historical data, add predictive analytics to your app analytics mix. It will help you unlock insights into future user behavior so you can adjust your product decisions for the best possible outcomes.
And if you aren’t collecting any data on your app’s revenue yet, add a revenue platform to your app now. If you have a subscription app, check out Adapty for this, as Adapty can start giving you predictive analytics in as little as a week. Also, the analytics engine keeps improving its predictions as data flows in. Read more about Adapty’s predictive analytics here.
And remember that while predictive analytics is a big part of understanding where your app might be headed, it’s also essential to consider external factors that may need to be captured in its forecasting.
Recommended posts